Understanding the 3Vs of Big Data: Volume, Velocity, Variety
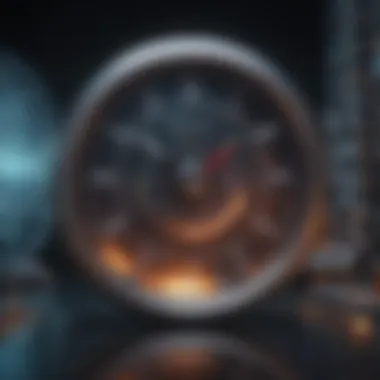
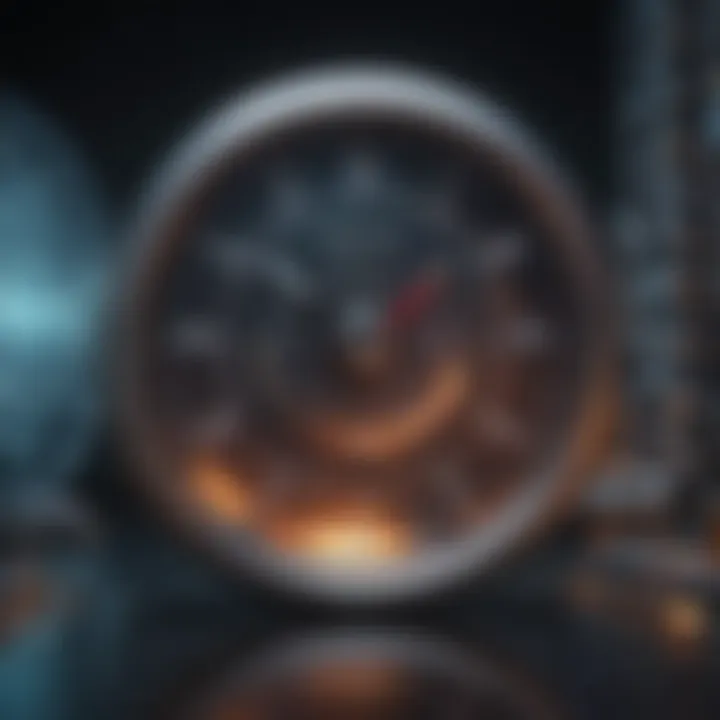
Intro
In today’s world of data, the conversation often centers around the three crucial elements known as the 3Vs: Volume, Velocity, and Variety. These factors act like the backbone of Big Data, influencing how organizations gather, store, analyze, and utilize vast quantities of information. Amidst the explosion of data generated daily, understanding these principles not only guides data management strategies but also illuminates potential opportunities and challenges across various fields.
The rise of social media, IoT, and advanced analytics has further amplified these dimensions, creating a complex landscape that demands meticulous navigation. With information overload becoming commonplace, organizations need to sift through significant volumes of data at rapid speeds, all while ensuring a diverse array of data types is effectively integrated into their systems.
As we break down each of these 3Vs, it’s essential to grasp their individual implications and how they interconnect to form a holistic view of the data ecosystem. This exploration aims to equip researchers and professionals with the insights needed to thrive in the modern data landscape.
Summary of Objectives
This article provides a detailed examination of the 3Vs—Volume, Velocity, and Variety—common challenges faced in these realms, and practical methodologies to tackle them. Each section will delve into what these terms mean in the context of Big Data and why they matter so greatly in decision-making processes.
Importance of the Research
Understanding the significance of these three dimensions serves not just as a theoretical exercise. It is about unlocking opportunities for innovation and efficiency, informed decisions based upon accurate data analysis, and the potential pitfalls that those new to the scene may encounter. The relevance stretches across sectors from healthcare to finance, manufacturing to marketing. By understanding these key components, organizations can position themselves to get the most out of their data initiatives.
Results and Discussion
Presentation of Findings
As we strive to uncover the layers within these 3Vs, let’s break them down:
- Volume: This aspect speaks to the sheer amount of data being generated. From customer interactions to transaction records, the scale is monumental. Organizations may collect terabytes or even petabytes of information. For example, Facebook handles over 100 billion messages sent each day, highlighting the need for steadfast data management practices.
- Velocity: Referring to the speed at which data flows, velocity influences how quickly organizations can respond. The instantaneous nature of social media means trends can shift on a dime. In such cases, real-time analytics tools are essential to capture and act upon data swiftly, converting insight into action before an opportunity is lost.
- Variety: This component deals with the diversity of data types—structured, semi-structured, and unstructured. Organizations face the task of harmonizing various data sources to provide a comprehensive picture. Think of the mix that includes text, images, videos, and sensor data. Each presents its own set of challenges in integration and analysis.
Implications of Results
The interplay between these three factors is profound. For example, a robust data strategy needs not only to handle large volumes but also to analyze them alongside varied data formats in real-time. The challenge here is significant; poor integration can lead to misleading insights, undermining strategic decisions.
Conversely, mastering the 3Vs opens up a wealth of possibilities. Companies can optimize operations, enhance customer experiences, and drive innovation in ways previously deemed impossible. As such, businesses willing to embrace these complexities are more likely to sprint ahead in their fields.
"Navigating the intricacies of Big Data is like steering a ship through changing tides. Without understanding the flows of Volume, Velocity, and Variety, one risks capsizing in a sea of information."
Through this detailed exploration of the 3Vs, the article invites readers to look deeper into their practices. By doing so, one might uncover new strategies to leverage data, transforming it from a mere byproduct of operations into a core asset that drives success.
Prologue to Big Data
In the ever-evolving landscape of technology, understanding Big Data is no longer optional. It's integral for anyone wanting to glean insights from the vast oceans of information generated daily. Big Data encompasses not just the sheer amount of data collected but also the speed at which it is generated and its diverse forms. Recognizing these elements—Volume, Velocity, and Variety—sets the stage for more informed discussions about data strategies and analytics. This article serves as a compass for navigating the complex world of Big Data, illuminating the critical role it plays in research, business, and beyond.
Definition of Big Data
Big Data refers to datasets that are so large, fast, or complex that they become challenging to process using traditional methods. The data can come from various sources, such as social media posts, transactional records, or sensor data from connected devices. In a nutshell, if you can’t handle it with regular software tools, it likely falls under the umbrella of Big Data. This complexity demands specialized technologies and approaches to analyze and harness its potential effectively. The importance of understanding this definition helps in appreciating the implications of strategic data analysis.
Historical Context
Peeking into the history of Big Data reveals an interesting journey. The term itself gained traction in the early 2000s, influenced by advances in storage solutions and computing power. Prior to this era, organizations dealt with relatively small datasets, often managed on spreadsheets. The shift began when companies like Google and Amazon started exploiting their user data for better business decisions. This revolution marked the start of an age where data became the new gold, bullion sought after by every sector, from healthcare to finance.
Big Data's roots can also be traced back to older concepts such as data warehousing and business intelligence. They laid the groundwork but lacked the agility to handle today's massive datasets. The introduction of frameworks like Hadoop in 2006 introduced a game-changing ability to skip the bottleneck of traditional data processing. With these advancements, the Big Data phenomenon morphed into a critical component of strategic planning and operational efficiency in organizations.
"In the age of information, not knowing how to deal with data is like searching for treasure without a map."
Understanding Big Data is not merely about grasping definitions or historical backgrounds; it's about recognizing the profound impact it can have on decision-making and organizational performance. As we dive deeper, the 3Vs provide a framework to explore the volume, velocity, and variety that define this burgeoning field.
Understanding the 3Vs
Understanding the 3Vs of big data is crucial for mastering modern data analytics. These elements—Volume, Velocity, and Variety—not only define the landscape of big data but also dictate the strategies employed for managing and analyzing it. Ignoring any one of these aspects can lead to incomplete insights and hinder decision-making processes across various industries.
Overview of the 3Vs
- Volume refers to the sheer amount of data generated. In today’s world, terabytes to petabytes of information are collected every day, from consumer behavior to sensor data.
- Velocity signifies the speed at which data is generated and analyzed. In sectors like finance or healthcare, where time is of the essence, rapid data processing can mean the difference between a successful outcome and catastrophic failure.
- Variety speaks to the diverse forms of data, which can be structured, unstructured, or semi-structured. For instance, social media posts, transaction records, and IoT sensor data all come from different sources yet are relevant in building a comprehensive picture.
Understanding these 3Vs allows organizations to harness information effectively. When businesses take this holistic view, they can devise comprehensive strategies that improve efficiency, uncover trends, and forecast future outcomes.
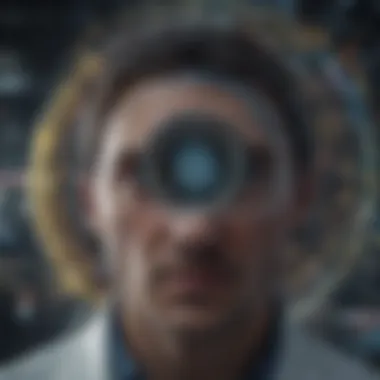
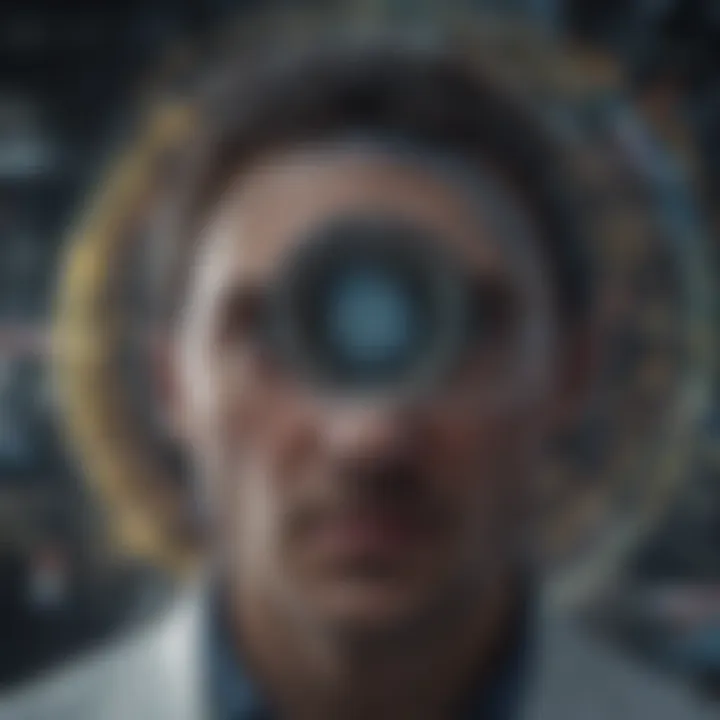
Importance in Data Analytics
The importance of the 3Vs in data analytics cannot be overstated. Organizations that recognize the interplay between Volume, Velocity, and Variety are better positioned to leverage their data.
- Enhanced Decision-Making: When managers have timely insights from massive datasets, they can make more informed decisions that consider multiple data perspectives.
- Operational Efficiency: By understanding the volume of data, companies can optimize their processes. For example, they might improve data storage solutions or enhance resource allocation.
- Risk Management: Rapid analysis of large, diverse datasets can help mitigate risks. For instance, banks utilizing real-time data analytics can detect fraudulent transactions before any significant damage occurs.
- Innovative Solutions: Diverse data types inspire creative analytics methods. Companies can mix conventional structured data with social media commentary to generate actionable insights that wouldn't emerge from a single data source.
Volume: The Scale of Data
In the vast landscape of Big Data, Volume serves as the cornerstone metric, distinguishing it from traditional data environments. The sheer magnitude of data generated today plays a crucial role, not only in informing decisions but also in shaping analytics methodologies. It influences storage strategies, processing capabilities, and ultimately, the insights gained from this ocean of information. When we talk about volume, it’s akin to standing in front of a massive tidal wave—understanding its size is key to harnessing its power.
Defining Volume
Volume can be understood as the amount of data collected, stored, and processed. In practical terms, it refers to the gigabytes, terabytes, or even petabytes of data that organizations must contend with on a daily basis. This extensive quantity can stem from various operational sources—everything from customer transactions, social media interactions, to Internet of Things (IoT) devices generating streams of data at an unprecedented rate. For organizations reinventing business strategies, grasping the concept of volume is paramount, as the insights extracted depend significantly on the amount of data at hand.
Sources of Big Data Volume
When discussing sources of data volume, one must acknowledge a diverse array of channels that continuously feed into data warehouses:
- Transactional data, which includes sales records and inventory management from retail platforms.
- Social media activity, representing millions of tweets, posts, and interactions, often in real-time.
- IoT devices, which produce continuous streams of data. For example, smart thermostats constantly send information about temperature settings and energy usage.
- Sensor data from industrial machinery that logs performance metrics enhancing operational efficiency.
Every interaction and transaction contributes, and understanding these sources helps organizations tap into the potential insights waiting to be discovered.
Challenges Associated with High Volume
However, the journey into high data volume isn’t without its tripwires. Organizations face multifaceted challenges when handling large-scale data.
Infrastructure Limitations
Infrastructure limitations often pose a significant hurdle. Many existing systems struggle to accommodate the vastness of data influx. For example, if a business relies on outdated servers, scaling up to handle increased data loads can be costly and technically complex. A key characteristic of this limitation is scalability—the ability of the infrastructure to grow with data needs. Systems that are not designed for scalability can become bottlenecks.
The unique feature here is that investing in scalable solutions can lead to operational agility. Businesses that anticipate volume growth and invest in cloud solutions or elastic storage options often find themselves better positioned to leverage data insights.
Data Storage Solutions
When addressing data storage solutions, organizations must consider both costs and accessibility. Traditional storage methods may no longer suffice. Modern approaches include cloud storage solutions like Amazon S3 or Google Cloud Storage, which offer scalability and durability. The key characteristic of these solutions is their on-demand flexibility. This means organizations can pay for storage as needed rather than over-committing to physical server space.
While cloud storage offers convenience and adaptability, it can also introduce risks, like potential downtime or the need for robust cybersecurity measures to safeguard sensitive information.
Processing Demands
As data volume escalates, so do the processing demands. This ties back to the infrastructure and storage challenges, but it is also about the speed and efficiency of data processing. Organizations are often tasked with employing advanced computing techniques, such as artificial intelligence and machine learning, to keep pace with data analytics. A crucial characteristic of processing demands is real-time processing, which allows organizations to derive insights instantaneously.
But real-time processing can be resource-intensive, requiring substantial computational power and skilled personnel. Therefore, businesses must carefully evaluate their processing capabilities and ensure they possess the right tools for simultaneous data handling without ground to a standstill.
"Navigating the volume of data is akin to trying to drink from a fire hose; it requires strategic planning and robust capabilities."
In summary, while high data volume presents exciting opportunities for insights and growth, it also requires organizations to reassess their infrastructure, storage options, and processing methodologies. Recognizing these challenges and addressing them effectively is vital for harnessing the true power of Big Data.
Velocity: The Speed of Data Generation
Understanding the velocity of data is crucial in the realms of big data. It refers to the pace at which data is created, processed, and analyzed. With the continuous influx of information from various sources such as social media, sensors, and online transactions, the ability to harness this rapidly flowing data is more important than ever. In essence, velocity is not just about speed; it embodies the challenges and opportunities that arise as data comes pouring in.
Defining Velocity
Velocity encompasses the rapid generation and processing of information. It highlights how swiftly data can be collected and transformed into actionable insights. In today's digital landscape, data doesn't trickle in—it floods in. The capacity to manage and analyze this torrent of data requires sophisticated tools and strategies. The faster an organization can pipeline data into its analytics processes, the better its performance in decision making and strategy development.
Factors Contributing to Data Velocity
Several factors support the speed of data generation:
- Internet of Things (IoT): Devices connected to the internet are buzzing with activity, generating data at lightning speed. Everything from smart home devices to industrial machinery contributes to this vast ocean of information.
- Social Media: Platforms like Twitter and Facebook keep users posting, sharing, and reacting in real time, resulting in a constant flow of new data insights.
- E-commerce Transactions: With the surge in online shopping, data from customer interactions—likes, purchases, reviews—comes in quickly and is voluminous.
- Surveillance Systems: Modern security cameras with advanced sensing technology create streams of data instantly, requiring timely analysis for effective monitoring.
Implications of Rapid Data Flow
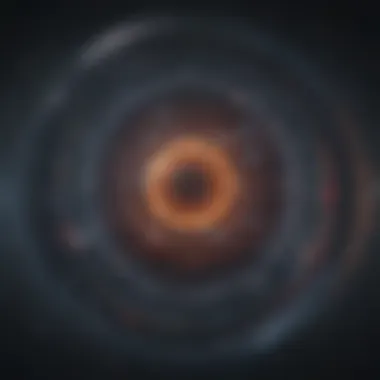
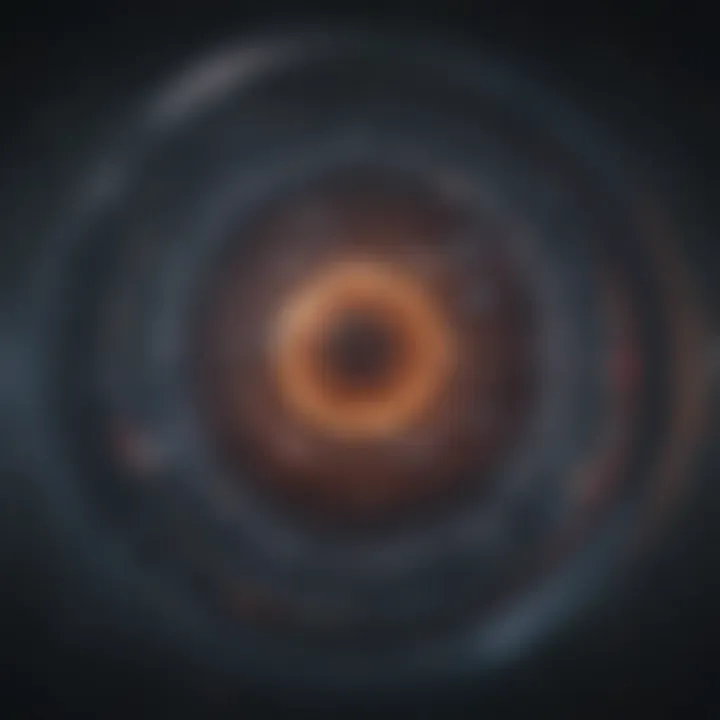
The ability to navigate the rapid pace of data flow carries significant implications:
Real-Time Analytics
Real-time analytics is at the forefront when it comes to the advantages of data velocity. This approach enables companies to analyze data as it becomes available. A key characteristic of real-time analytics is its ability to support immediate decision-making. It often integrates sophisticated algorithms and technologies. The unique feature of this approach lies in its responsiveness; businesses can pivot quickly based on real-time data insights. However, the challenges include the need for robust infrastructure and the potential for information overload, which can muddy decision-making processes.
Operational Challenges
Amid the benefits, operational challenges can rear their heads in the fast-paced data world. Managing the influx of data often demands more resources, which can be a sticking point for many organizations. A primary characteristic of these challenges is the requirement for constant monitoring and updating of data management practices. Organizations must stay prepared to prevent their systems from becoming bogged down. The unique feature here is the balance between speed and resource allocation; while it is great to have fast data, the infrastructure must be capable of handling it effectively, which sometimes strains technical capacities.
Decision-Making Impact
The impact of data velocity on decision-making cannot be overstated. With swift access to information, stakeholders can make informed choices that capitalize on current trends. Its key characteristic lies in its influence; decisions can be data-driven rather than gut-feeling based. A distinctive aspect here is the ability to predict trends based on real-time data. However, while rapid decisions can lead to competitive advantages, it can also prompt hasty conclusions based on incomplete data, potentially leading to misalignment with long-term strategies.
"In the age of data, speed is a pivotal currency that influences survival and growth in modern business landscapes."
By understanding the nuances of data velocity, organizations can better position themselves to leverage its potentials while navigating the accompanying challenges.
Variety: The Diversity of Data Forms
Understanding the variety within Big Data is akin to navigating a sprawling library with countless genres and sub-genres. In this context, variety refers to the many different forms data can take, and each form offers its own unique benefits and challenges. This multifaceted nature not only enriches analysis but also poses significant hurdles in data management. Different categories of data—structured, unstructured, and semistructured—allow organizations to glean insights from diverse sources, enhancing decision-making processes across various sectors.
Defining Variety
When we talk about variety in data, we hint at the heterogeneity of the information we gather. It encapsulates differences in data formats, structures, and source origins. These aspects intertwine to create a rich tapestry of data that can be used in various analytical frameworks. It's not just about having a lot of data; it’s about having the right kind of data to ask meaningful questions and find useful answers. This understanding is pivotal for any organization striving to stay relevant in an ecosystem increasingly defined by information.
Types of Data in Big Data
Structured Data
Structured data is like a well-organized filing cabinet. It appears in neatly arranged tables, making it easy to access and analyze. This type of data is usually quantitative and resides in relational databases. Think of customer information collected through forms—name, address, purchase history—in a straightforward format that allows for quick queries. Its key characteristic lies in its format; it follows a pre-defined data model, which often makes it the go-to choice for businesses aiming for efficiency in data retrieval and analysis. However, while it shines in organization, it lacks the depth of insight that more informal, unstructured data can provide.
Unstructured Data
In contrast to structured data, unstructured data is the chaotic creative expression of information. This category includes emails, social media posts, videos, and documents without a uniform format. The beauty of unstructured data lies in the richness of the context it provides. For instance, analyzing customer feedback on social platforms can unveil sentiments and trends that traditional surveys might miss. Yet, it poses a significant challenge: without a clear structure, it can be time-consuming and resource-intensive to extract meaningful insights. Therefore, while it represents vast potential, organizations must adopt advanced analytics and machine learning tools to make sense of this complexity.
Semistructured Data
Somewhere between structured and unstructured lies semistructured data. This type is like a billboard that provides information but lacks a definite form. Examples include XML and JSON files, where data is organized but does not fit neatly into tables. Semistructured data allows for a degree of flexibility, enabling organizations to identify patterns without the constraints of strict structure. However, the challenge here is ensuring data quality and integrity, as the lack of a rigid format may lead to inconsistencies. Proper management of this data type is crucial as it can offer valuable insights without the complete chaos of unstructured data.
Challenges in Managing Diverse Data Types
Integration Issues
Handling multiple types of data can lead to integration issues, which often emerge as data silos within organizations. Different departments might have their own systems, making it difficult to get a cohesive view of the data landscape. This fragmentation can hinder decision-making, as teams may miss critical insights hidden in other data types. Finding a solution that allows for seamless integration across various data formats is essential to harness the full potential of Big Data.
Data Quality Concerns
Achieving high data quality in a variety-rich environment is no walk in the park. Inconsistent formats and varying source credibility can result in inaccuracies that skew analysis. Most organizations focus on quantity, but poor quality data can lead to misguided decisions. Hence, employing robust data governance frameworks is crucial to maintaining quality, reliability, and trustworthiness in analytics.
Analytical Complexity
Finally, the diversity of data types introduces analytical complexity. With structured, unstructured, and semistructured data coexisting, analytical processes can become convoluted. Teams must navigate the intricate dynamics of extracting and interpreting insights from varied sources, often requiring specialized skills or tools. While the complexity can be daunting, it also represents an opportunity for innovation—figuring out new methods to extract value from the chaos can lead to groundbreaking discoveries and more informed decision-making across the board.
"In a world where data is the new oil, those who master the various forms of data will reign supreme."
In summary, embracing the variety inherent in Big Data is crucial for realizing its full potential. Recognizing the different data forms and their implications can significantly shape the analytics landscape and drive meaningful outcomes for organizations.
The Integration of the 3Vs
The concept of interweaving Volume, Velocity, and Variety stands at the heart of comprehending the magnitude of Big Data. Each component does not operate in a vacuum; rather, they are inherently linked, influencing one another in complex ways. The integration of the 3Vs is crucial not only for data analysis but also for dictating strategies that shape digital landscapes across various industries. When these three aspects converge, they generate a rich tapestry of insights that can drive informed decision-making and enhance operational efficiencies.
One of the primary benefits of recognizing the interplay among the 3Vs is illustrated through enhanced predictive capabilities. For instance, a business that effectively manages high-volume data at rapid speeds while incorporating diverse data types can better foresee market trends, consumer behavior, and operational challenges. This synchronization can lead to a deeper understanding of the market's pulse, allowing companies to adjust their strategies swiftly and effectively.
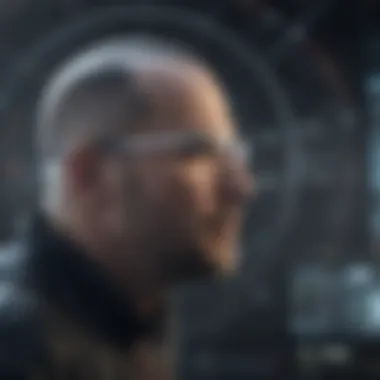
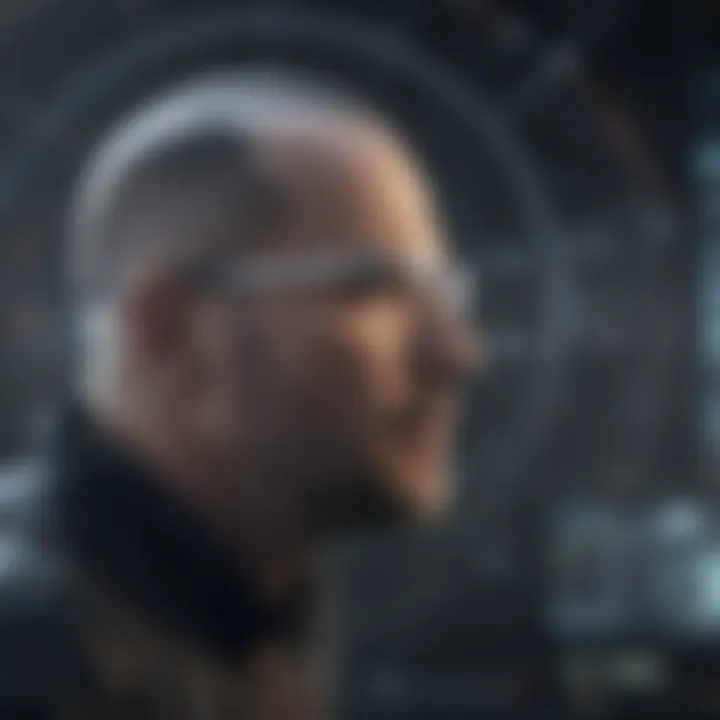
However, integration is not without its challenges. As the volume of data grows exponentially, maintaining velocity becomes increasingly complex. A business may find itself overwhelmed if it lacks the infrastructure to process vast amounts of data quickly. The variety of data compounds this issue since different data types require different handling techniques. Thus, an integrated approach necessitates a comprehensive understanding of each component's impact on the others.
Interdependencies Among the 3Vs
Understanding the interdependencies among the 3Vs leads to a more nuanced approach in data handling. For example, when discussing Volume, three key considerations emerge:
- Processing Power: A substantial amount of data necessitates advanced processing capabilities. If a business cannot process high volume fast, it impedes effective analysis.
- Storage Solutions: Storing large datasets often presents challenges, particularly when Variety is introduced. Hybrid storage solutions may be necessary to accommodate structured, semi-structured, and unstructured data effectively.
- Data Quality: High data volume can lead to data quality issues. Ensuring quality while maintaining speed (velocity) is paramount.
In a real-world context, think of a social media platform like Facebook. They routinely process millions of posts and interactions per second, representing Velocity. This rapid influx of data needs to be managed while ensuring it remains Variety-rich—from text posts to images and videos. The sheer Volume of this data calls for robust data architecture capable of real-time processing.
Case Studies Illustrating Integration
To illustrate the integration of the 3Vs, consider the case of Netflix. The company not only analyzes vast amounts of data regarding user behavior but does so at breakneck speed to deliver personalized recommendations, offering a classic example of effective Volume-Velocity integration.
Moreover, their users submit ratings, reviews, and feedback in varied formats—textual, visual, etc.—showing the impact of Variety alongside Volume and Velocity. This integration allows Netflix to refine its recommender system continuously, showcasing how harnessing all three Vs can lead to enhanced user engagement.
In contrast, let's look at a traditional retail store that struggles with these aspects. It collects data through a point-of-sale system (Volume) but may lag in real-time processing (Velocity), leading to missed opportunities for timely analyses about inventory needs or customer purchases. The data might also be limited to structured sales data, lacking the diversity of types (Variety) that could inform promotional strategies.
This juxtaposition of cases underscores the importance and potential of successfully integrating the 3Vs. By fully embracing and understanding each aspect, organizations can position themselves to leverage data as a strategic asset, paving the way for innovation and sustained competitive advantage.
"The effective integration of Volume, Velocity, and Variety requires not only a robust tactical framework but also a visionary approach to data strategy."
By doing so, not only will they overcome the challenges posed by each V, but they will also uncover a wealth of opportunities previously hidden in the data they collect.
Future Trends in Big Data
As we peer into the horizon of Big Data, it becomes painfully clear that our current landscape is just the tip of the iceberg. Future trends in this realm promise not only to refine the tools we employ today but also to reshape the very fabric of data management and utilization. With the accelerating pace of technological advancements, understanding these trends will be crucial for students, researchers, educators, and professionals alike. By grasping what's on the horizon, these stakeholders can better prepare themselves to unlock the full potential of Big Data.
Emerging Technologies
The cutting-edge technology landscape is alive with possibilities that cater specifically to the growing complexities of Big Data. A few key players stand out:
- Artificial Intelligence and Machine Learning: These tools have already started to integrate into data processing and analysis, but their future potential seems boundless. Expect solutions that not only analyze data at lightning speed but can also learn from it, adapting processes in real-time.
- Distributed Computing: Consider platforms such as Hadoop and Apache Spark, which allow for processing vast amounts of data across multiple systems. As these technologies evolve, they enable more efficient ways to manage data loads, thereby reducing bottlenecks in processing.
- Edge Computing: With the rise of the Internet of Things, data collection is happening closer to where the data is generated. This trend shifts the need from traditional cloud computing systems to edge computing alternatives, where data is processed on local devices.
These technologies not only promise efficiency but also the capability to handle an almost overwhelming flow of data. As they develop, the implications for Volume, Velocity, and Variety grow substantially, setting the stage for more intricate data strategies.
Predictions for Big Data Evolution
Looking forward, several predictions may offer insights into how Big Data will transform further:
- Increased Focus on Data Privacy: With an avalanche of data comes the critical concern of data protection. Future regulations and more conscientious practices surrounding user privacy will shape how data is collected and used.
- Integration of Quantum Computing: Though still largely theoretical, quantum computing could revolutionize data analytics by allowing tasks that are currently computation-heavy to be executed almost instantaneously.
- Data Democratization: As tools become more user-friendly, the barrier to entry for utilizing Big Data will lower. This democratization means that more individuals and smaller organizations can engage with data analytics, unleashing creativity and innovation.
- Real-Time Decision Making: Businesses will increasingly rely on instant data findings using advanced predictive analytics, enabling them to react swiftly to market changes.
"The future of Big Data isn't about more data; it’s about making intelligent decisions and deriving insights faster than ever before."
Implications extend beyond mere technology—core business strategies will evolve alongside these shifts. Thus, staying attuned to these trends enables researchers and professionals to not only anticipate change but also align their methodologies accordingly. It's an ever-evolving dance between data, technology, and human insight, one that demands readiness for what lies ahead.
Closure: The Importance of the 3Vs
The 3Vs of Big Data—Volume, Velocity, and Variety—are not just an academic concept; they are fundamental to the modern world of data analytics. Understanding these three dimensions is critical for anyone who navigates or utilizes data in any capacity. They shape data strategies across industries and help to foster innovative approaches that enhance decision-making processes.
Summarizing Key Insights
The exploration of the 3Vs reveals distinct yet interlinked characteristics of data that professionals must grasp.
- Volume highlights the sheer amount of data generated, emphasizing the need for robust storage solutions and computational power. Without managing volume, organizations may find themselves overwhelmed and unable to extract meaningful insights.
- Velocity speaks to the rapid generation and processing of data. The pace at which information flows requires real-time analytics to stay competitive. Ignoring velocity can lead to missed opportunities or sluggish responses to market changes.
- Variety emphasizes the various forms data can take, from structured databases to unstructured social media posts. This diversity complicates data integration but also enriches the information pool, providing a more holistic view when analyzed correctly.
"In the information age, data is the new oil, but only if it is refined properly."
These insights underline why organizations need to prioritize their understanding of the 3Vs. The challenges they present are substantial, yet the opportunities they create are equally compelling. Embracing the complexity requires education, the right tools, and a willingness to adapt.
Implications for Researchers and Professionals
For researchers and professionals, recognizing the significance of the 3Vs sets the foundation for informed decision-making. With data becoming more accessible, understanding its characteristics can foster improved methodologies. Also, recognizing that data isn’t just about numbers but stories can lead to better insights and strategies.
- Research Focus: Academics are encouraged to push the envelope in exploring how to better handle large volumes of data while ensuring its integrity and quality.
- Professional Practices: On the ground, practitioners should advocate for tools and strategies that manage velocity, such as agile data processing methods, to keep up with fast-paced environments.
- Integration: Additionally, there's a pressing need for interdisciplinary collaboration, as combining insights from data science, statistics, and domain-specific knowledge enriches analysis and understanding.
The discussion around the 3Vs opens avenues for future research and collaboration among scholars and practitioners. Ensuring that data analytics grows in a way that benefits all sectors of society will require collective effort.
The ability to adeptly manage the 3Vs will likely distinguish leading organizations from those that struggle. As we continue to advance technologically, the symbiosis of Volume, Velocity, and Variety will remain critical in shaping the landscape of data analytics. Understanding this trinity equips professionals to make more informed choices, ensuring that they remain effective and competitive in an ever-evolving data-driven world.