Exploring Data Analytics Across Industries
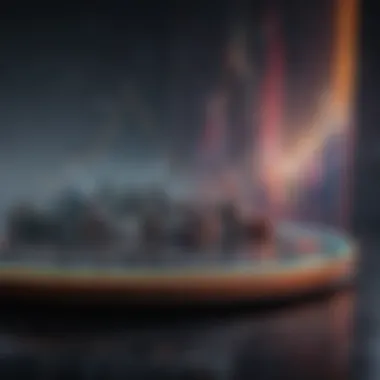
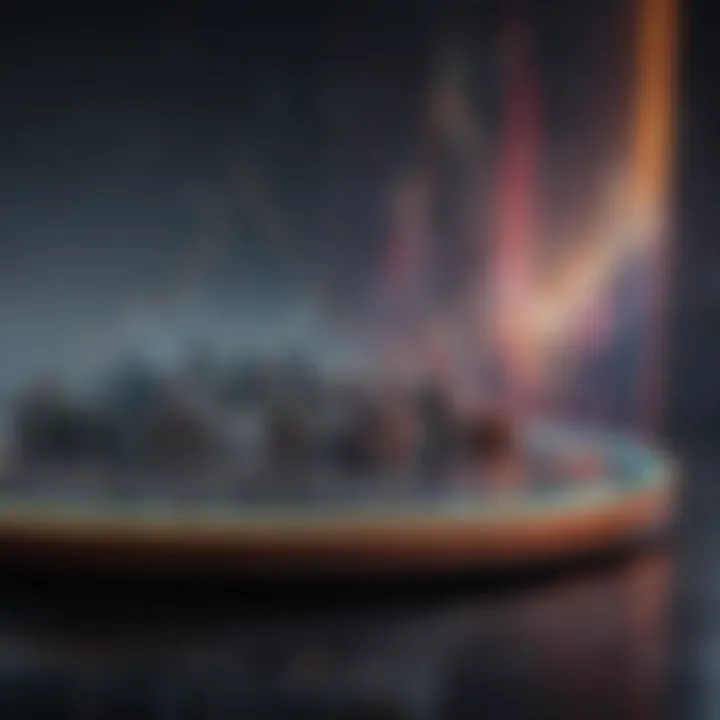
Intro
Data analytics has become a cornerstone of decision-making in various industries. As organizations face growing competition and a rapidly changing environment, the capacity to analyze data effectively is crucial. This evolving discipline encompasses methodologies aimed at deriving insights from large sets of data, ultimately enhancing operational efficiency and consumer engagement.
The application of data analytics spans numerous sectors, including healthcare, finance, retail, and manufacturing. Each of these industries utilizes data analytics to streamline processes, predict consumer behavior, and formulate strategies that foster growth. However, the process of integrating data analytics into existing systems presents several challenges. These include data quality, security concerns, and the need for a skilled workforce.
Understanding the fundamental principles of data analytics is vital for students, researchers, educators, and professionals alike. In this comprehensive overview, we will explore the objectives of data analytics, delve into its relevance in modern industry practices, and evaluate the implications of findings in the field.
By illuminating the transformational impact of data analytics, we aim to provide a clearer picture of how these practices shape the current and future landscape of various industries.
Prolusion to Data Analytics
Data analytics is a pivotal component in today's data-driven world. The ability to extract meaningful insights from vast amounts of information is not just a theoretical exercise but a fundamental business strategy. As industries evolve, understanding data analytics becomes increasingly vital to maintain a competitive edge. This section aims to provide a solid foundation on data analytics, introducing its definition and significance in various sectors.
Definition of Data Analytics
Data analytics refers to the systematic computational analysis of data. It encompasses the methods and tools used to inspect, clean, transform, and model data, with the goal of discovering useful information, suggesting conclusions, and supporting decision-making. The disciplines of data science and statistics play significant roles in this process.
Different types of data analytics exist, such as descriptive, predictive, and prescriptive analytics, each serving distinct purposes. Descriptive analytics provides insights into past performance, predictive analytics forecasts future outcomes, and prescriptive analytics recommends actions based on data analysis. Thus, data analytics is not merely about number crunching but about drawing actionable insights to guide business strategies.
Importance of Data Analytics in Industry
The importance of data analytics in industry cannot be overstated. It is an invaluable resource for enhancing operational efficiency, improving customer satisfaction, and driving innovation. Businesses leverage data to make informed decisions that align with market trends and consumer demands. Several key benefits are associated with the implementation of data analytics, namely:
- Enhanced Decision-Making: Data analytics provides the evidence needed to make informed choices, reducing reliance on intuition.
- Cost Reduction: Organizations can identify inefficiencies and optimize processes, leading to significant cost savings.
- Increased Revenue: By analyzing customer behavior and preferences, businesses can tailor their products and marketing strategies effectively.
- Risk Management: Data analytics helps identify potential risks and develop strategies for mitigation, ensuring resilience against unforeseen challenges.
"In the era of digital transformation, data analytics emerges as a vital tool for organizations aiming to succeed in a competitive landscape."
The integration of data analytics into business operations also fosters a culture of continuous improvement. Detail-oriented analysis not only reveals existing strengths but highlights areas requiring attention. As such, industries that embrace data analytics are better positioned to adapt and thrive in an ever-changing marketplace.
Types of Data Analytics
Understanding types of data analytics is crucial for leveraging data effectively in any industry. Each category of analytics serves a distinct purpose and offers unique insights that can drive decision-making processes. Organizations adopting these analytics can optimize operations, enhance strategies, and ultimately foster growth. The main types include descriptive, predictive, and prescriptive analytics.
Descriptive Analytics
Descriptive analytics plays a pivotal role in evaluating historical data to identify trends and patterns. It focuses on summarizing past events and providing clarity about what has happened. This type uses various statistical methods to transform raw data into meaningful insights.
Organizations benefit from descriptive analytics in several ways:
- Performance Measurement: Companies can assess the success of past initiatives through key performance indicators (KPIs).
- Trend Identification: Identifying trends helps in making informed decisions for future strategies.
- Data Visualization: Tools such as dashboards and reports present data in a user-friendly format, aiding decision-makers.
Descriptive analytics sets the stage for further analysis, leading to deeper insights into operational effectiveness.
Predictive Analytics
Predictive analytics goes a step further by forecasting future outcomes based on historical data. It incorporates advanced statistical techniques and machine learning algorithms to predict potential trends and behaviors. This form of analytics is valuable for anticipating customer needs and market changes.
Several advantages of predictive analytics include:
- Risk Assessment: Businesses can identify potential risks early and implement strategies to mitigate them.
- Customer Insights: Understanding customer behavior patterns aids in developing targeted marketing strategies.
- Resource Allocation: By predicting demands accurately, organizations can optimize resource allocation and reduce waste.
As industries evolve, predictive analytics become increasingly integral to making well-informed business decisions.
Prescriptive Analytics
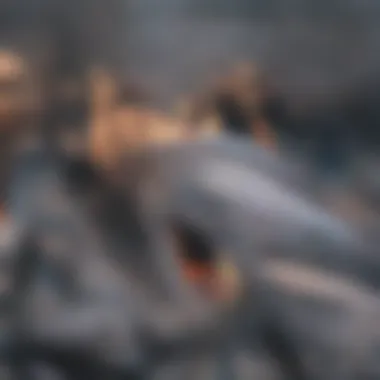
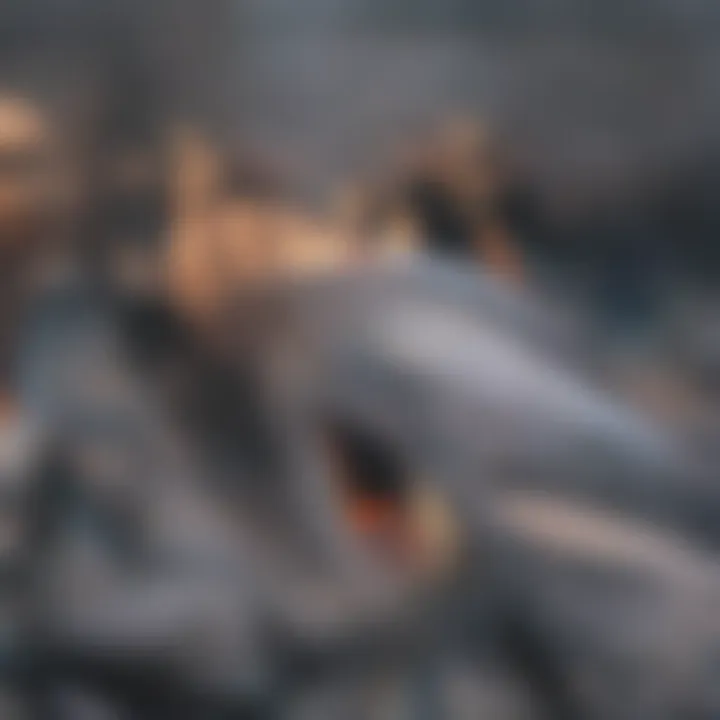
Prescriptive analytics is the most advanced type of data analytics. It not only predicts future outcomes but also provides recommendations on possible actions to take. This approach combines data with business rules, algorithms, and machine learning to suggest optimal decisions.
Key benefits of prescriptive analytics include:
- Optimal Decision-Making: Organizations can leverage these insights to make data-driven decisions, which improves efficiency.
- Scenario Analysis: The ability to analyze different scenarios allows organizations to prepare for various outcomes.
- Enhanced Strategy Development: Businesses can create tailored strategies based on specific recommendations from analytics.
The implementation of prescriptive analytics can significantly improve operational effectiveness, driving organizations towards achieving their objectives.
Applications of Data Analytics in Various Industries
The applications of data analytics across diverse industries underline its pivotal role in the modern business environment. Organizations harness insights from data to enhance processes, drive strategic decisions, and gain competitive advantages. As industries evolve, so too does the reliance on analytics to inform actions. Implementing data analytics not only optimizes workflows but also enriches understanding of customer behavior and market trends.
Data Analytics in Healthcare
Patient Care Optimization
Patient care optimization involves utilizing data analytics to improve the quality of care for patients. By analyzing patient records, healthcare providers can identify trends in patient health. This insight allows for tailored treatments that significantly enhance patient outcomes. By focusing on preventive care, hospitals can reduce readmission rates. This method is a popular choice as it helps healthcare providers allocate resources more effectively.
However, one disadvantage is the need for robust data governance to protect sensitive information.
Predictive Modeling for Disease Outbreaks
Predictive modeling stands out in its capacity to foresee potential health crises. It uses historical data to identify patterns that may predict outbreaks. This aspect is critical in planning and resource allocation for healthcare systems. Accurate models can identify at-risk populations and trigger timely interventions. However, the reliability of predictions heavily depends on the quality of input data, making ongoing data collection vital.
Operational Efficiency in Hospitals
Operational efficiency is vital for hospitals aiming to deliver high-quality care while managing costs. Data analytics helps streamline processes like patient admission, bed management, and staff scheduling. This efficiency improves resource utilization and patient flow within the hospital. Healthcare facilities, therefore, can provide timely care, which is critical for patient satisfaction. Nevertheless, implementing such systems can require substantial time and investment.
Data Analytics in Finance
Risk Management
In finance, risk management is fundamental for ensuring stability and growth. Data analytics aids in identifying and analyzing financial risks associated with investments and market fluctuations. By leveraging predictive models, financial institutions can forecast potential downturns. This proactive approach allows organizations to create strategies to mitigate losses. Nonetheless, reliance on historical data may limit the scope of predictions.
Fraud Detection
Fraud detection significantly benefits from data analytics by identifying unusual patterns and behaviors in transactions. Financial institutions deploy real-time analytics to detect fraud patterns, ensuring a swift response. Early detection helps mitigate losses and protect consumer trust. However, false positives can inconvenience customers, leading to a need for balance between security and user experience.
Investment Strategies
Investment strategies are shaped by data analytics, providing investors insights into market trends and individual securities. Using analytics, investors can make informed decisions based on analytics of past performance and projections. This richness of data helps in diversifying portfolios to minimize risks. However, over-reliance on analytics might lead to neglecting fundamental market principles.
Data Analytics in Manufacturing
Supply Chain Management
Effective supply chain management is critical for manufacturers aiming to maximize efficiency. Data analytics enables real-time tracking of inventory and logistics, optimizing supply chain operations. This data-driven approach ensures timely delivery and reduces waste. Enhanced visibility across the supply chain can significantly improve operational performance. The downside, however, lies in the complexity of integrating disparate data sources.
Predictive Maintenance
Predictive maintenance involves analyzing data from equipment to foresee failures before they occur. This strategy can save manufacturers significant costs associated with unplanned downtimes. By anticipating maintenance needs, companies ensure continuous production flow. However, the implementation of predictive maintenance technologies can be costly and require skilled personnel.
Quality Control
Quality control is enhanced through data analytics by identifying defects in products during the manufacturing process. By monitoring production data continuously, manufacturers can address quality issues in real-time. This aspect not only promotes higher product standards but also boosts customer confidence. A potential disadvantage is the need for substantive initial investments in monitoring technology and analytics systems.
Data Analytics in Retail
Customer Segmentation

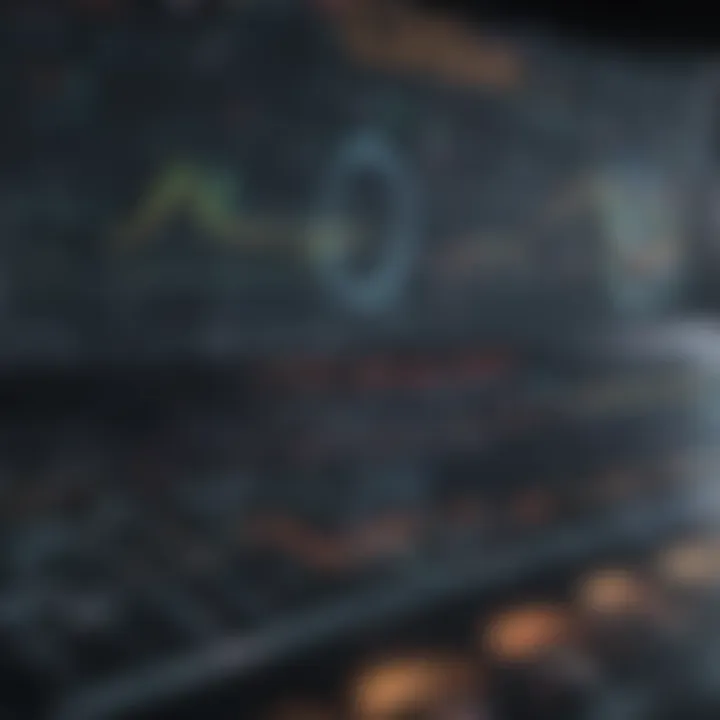
Customer segmentation involves dividing a customer base into groups for targeted marketing. Analytics provides insights into buying patterns, preferences, and behaviors. Retailers can personalize marketing efforts, enhancing customer engagement. However, improper segmentation can lead to misallocation of marketing resources and poor customer experiences.
Inventory Management
Inventory management benefits immensely from data analytics by optimizing stock levels. Analyzing sales data allows retailers to forecast demand accurately. This proactive approach minimizes overstock and stockouts, improving cash flow. But, reliance on data analytics can be misleading if the data does not capture real-time market changes.
Sales Forecasting
Sales forecasting incorporates data analytics to predict future sales based on historical data. Accurate forecasting can inform business strategies, promotional campaigns, and resource allocation. Retailers can optimize stock levels and avoid waste. Nevertheless, erratic market conditions can lead to inaccurate forecasts, calling into question the reliability of the data used.
The application of data analytics is crucial for industries striving to enhance their operational efficiencies and market positioning. By embracing analytics, organizations can make more informed decisions that ultimately drive success.
Methodologies Used in Data Analytics
Understanding the methodologies used in data analytics is essential for grasping how organizations derive insights from vast amounts of data. These methodologies provide frameworks that shape the way data is collected, processed, and interpreted. By employing various techniques, businesses can optimize operations, enhance decision-making, and drive innovation. Each methodology has its unique strengths and weaknesses, making it vital for professionals to choose the right one based on their specific needs.
Statistical Methods
Statistical methods play a foundational role in data analytics. They provide robust techniques for interpreting data and uncovering patterns. Basic statistical measures, like mean, median, and mode, help in understanding data distributions. More complex tests, such as regression analysis, enable analysts to explore relationships between variables. This understanding is crucial when performing predictive analytics, where past data informs future trends.
An important element of statistical methods is hypothesis testing. This process loads with significance as it allows researchers to validate their assumptions and conclusions. Properly applied statistical techniques can reveal actionable insights that may otherwise remain hidden. Moreover, decision-makers gain confidence in their strategies based on statistical evidence, minimizing risks associated with uncertainty.
"Effective use of statistical methods can turn raw data into actionable insights, guiding strategic decisions."
Machine Learning Techniques
Machine learning techniques revolutionize data analytics by automating complex analyses. Unlike traditional methods that require explicit programming, machine learning models learn from data patterns and improve over time. This adaptability is particularly beneficial as the data landscape grows continuously.
Supervised learning is one of the most common paradigms. In this approach, models are trained on labeled datasets, allowing them to classify new data points based on learned patterns. On the other hand, unsupervised learning works with unlabeled data, uncovering hidden structures without pre-defined categories. Both techniques contribute significantly to predictive analytics, enhancing accuracy in forecasting and trend identification.
The advantages of machine learning include greater efficiency and the ability to handle large datasets more effectively than human analysts. However, the complexity of building and maintaining these models can present challenges, including the need for expertise and specialized software.
Data Mining Approaches
Data mining approaches focus on discovering patterns and extracting valuable information from large datasets. These methods combine statistical analysis, machine learning, and database systems to analyze vast amounts of information. Data mining techniques include clustering, classification, and association rule mining.
Clustering helps group similar data entities into clusters, making it easier to identify trends. Classification, on the other hand, involves assigning data points to predefined categories based on attributes. This is particularly useful in sectors like marketing for targeted advertising. Association rule mining uncovers relationships among variables, revealing insights like purchasing patterns in retail data.
One primary consideration when applying data mining is the quality of data. High-quality data leads to better insights, while poor-quality data can lead to misleading conclusions. Additionally, organizations must keep ethical considerations in mind, ensuring that their data mining efforts respect user privacy and transparency.
Challenges in Implementing Data Analytics
The implementation of data analytics within industries comes with its own array of challenges that can impede progress and effectiveness. Addressing these challenges is critical to harnessing the full potential of data-driven decision-making. Thoughtful consideration of these issues can lead to enhanced operational efficiencies, improved customer insights, and sustainable competitive advantages. Understanding the complexity of these challenges enables organizations to formulate better strategies for overcoming them.
Data Privacy Concerns
With the increasing reliance on data analytics comes the pressing issue of data privacy. Companies often collect vast amounts of personal information to gain insights. This data collection must be conducted in compliance with various regulations such as GDPR and CCPA. Mishandling sensitive data not only risks legal repercussions but also erodes consumer trust.
Maintaining data privacy involves implementing robust security measures, including encryption and access controls. Additionally, organizations must educate their employees on best practices surrounding data management. Transparency with customers about how their data is used is also crucial in mitigating privacy concerns. Many businesses face a balancing act, striving to use data while ensuring that ethical standards and privacy laws are respected.
Integration with Legacy Systems
Another significant hurdle in the realm of data analytics is the integration with legacy systems. Many organizations have longstanding IT infrastructures that may not support modern data analytics tools. Legacy systems can be resistant to integration due to outdated technology or lack of compatibility with new software solutions.
The challenges here include data silos and loss of efficiency. Often, valuable data resides in disparate sources, making it difficult to harness it effectively. For organizations looking to implement data analytics, they must consider investing in middleware solutions or fully upgrading their systems. The transition can be costly and time-consuming, further complicating the implementation process. Successful integration requires careful planning and execution to ensure that existing operations are minimally disrupted.
Skill Shortage in Data Science
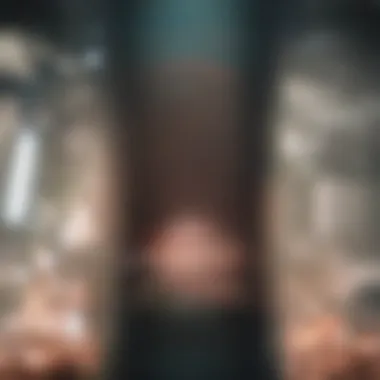
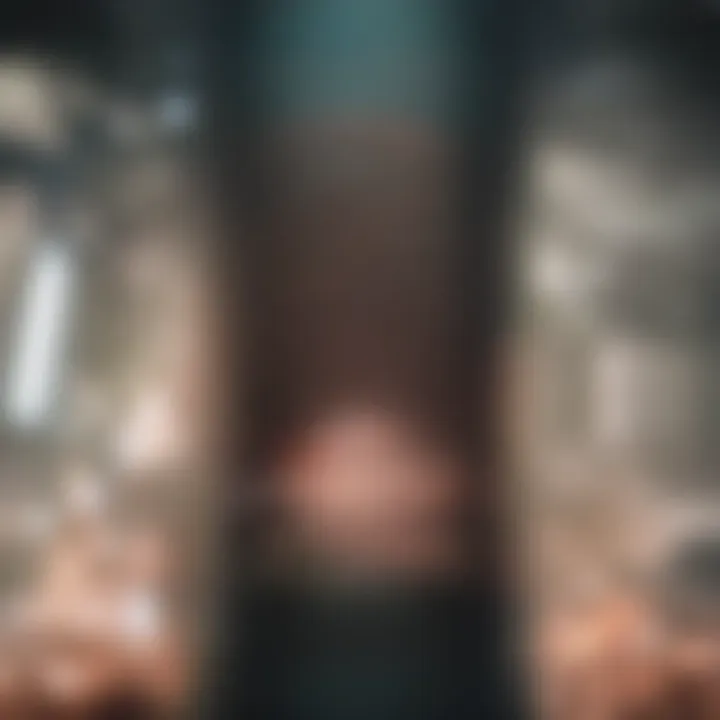
Finally, the skill shortage in data science remains a formidable challenge for many industries. Even though the demand for data analytics professionals has surged, there are insufficient numbers of qualified individuals to fill these roles. Many companies struggle to find data scientists with the right blend of analytical skills, domain expertise, and technical knowledge.
Organizations may need to invest in training programs for existing employees or look into partnerships with educational institutions to foster a new generation of data professionals. This not only helps bridge the skill gap but also encourages a data-driven culture within the organization. Developing talent internally can be a valuable strategy for overcoming personnel shortages in the data science field.
"The challenges in implementing data analytics are complex but essential to address to fully leverage data's potential in driving informed business decisions."
In summary, addressing data privacy concerns, integrating with legacy systems, and overcoming the skill shortage are crucial steps in the successful implementation of data analytics in industry. By acknowledging and strategizing around these challenges, organizations can unlock the value of data analytics for enhanced decision-making and operational effectiveness.
Future Trends in Data Analytics
Data analytics is continuously evolving, shaping how industries operate. Understanding the future trends is essential for organizations aiming to remain competitive. This section discusses three prominent trends: Artificial Intelligence and Automated Analytics, Real-Time Analytics, and Ethical AI in Data Analytics. These trends provide insights into evolving methodologies and practices in the data analytics landscape.
Artificial Intelligence and Automated Analytics
Artificial Intelligence (AI) significantly enhances data analytics processes. AI algorithms automate data collection, cleansing, and even preliminary analysis. This creates faster and more efficient workflows, allowing companies to derive insights quickly.
The benefits of integrating AI include:
- Increased Efficiency: Automation reduces the time spent on repetitive tasks, freeing up analysts to focus on more complex analytical tasks.
- Enhanced Accuracy: AI can identify patterns and anomalies that may go unnoticed by human analysts.
- Scalability: As the volume of data grows, AI systems can efficiently process large datasets without a proportional increase in resources.
However, there are considerations to keep in mind:
- Companies must ensure the AI models' decisions are transparent and understandable.
- Ongoing supervision is needed to ensure the AI continues to align with business goals.
Real-Time Analytics
As the pace of business accelerates, real-time analytics becomes increasingly critical. This practice involves the continual input of data and immediate processing, allowing organizations to make timely decisions based on current information.
Benefits include:
- Immediate Insight Generation: Real-time data provides actionable insights immediately, supporting operational decision-making.
- Improved Customer Interaction: Businesses can respond to customer actions as they happen, enhancing the customer experience.
- Proactive Management: Organizations can implement preventative measures before issues escalate, reducing disruptions.
Despite its advantages, challenges include the need for robust infrastructure capable of handling continuous data streams and ensuring data integrity in fast-paced environments.
Ethical AI in Data Analytics
As data utilization expands, ethical considerations become paramount. Ethical AI focuses on the responsible use of data analytics within ethical frameworks. This trend emphasizes fairness, accountability, and transparency in AI algorithms.
Key components include:
- Bias Mitigation: Organizations must actively work to identify and eliminate biases in AI models to ensure equitable outcomes.
- Data Privacy: Respecting user privacy and data protection laws is crucial. Companies need to handle personal data with care.
- Transparency: Providing clarity on how AI models operate and make decisions is essential for building trust with users.
In summary, the intersection of AI and data analytics brings forward exciting prospects. However, it also presents significant challenges. Businesses should think critically about the future trends in data analytics and develop strategies that prioritize ethics and effectiveness.
Culmination
This article has explored the multifaceted role of data analytics in industry, offering insights into its applications, methodologies, challenges, and its future trajectory. The conclusion acts as a synthesis of the key points discussed throughout the paper, providing clarity about the significance of these elements. Data analytics is no longer a supplementary aspect of business practices; it is central to informed decision-making and operational strategies. Understanding how to leverage data effectively can lead to improved efficiency, enhanced consumer understanding, and ultimately better outcomes for companies in various sectors.
Summary of Key Points
The key takeaways from this investigation include:
- Evolving Role: Data analytics continues to evolve within industries, integrating advanced technologies like machine learning and artificial intelligence.
- Widespread Applications: Applications are shown in diverse fields such as healthcare, finance, manufacturing, and retail, each demonstrating unique benefits tailored to specific needs.
- Methodologies: The methodologies employed range from basic statistical methods to complex machine learning techniques, emphasizing a need for skilled professionals capable of navigating these tools.
- Challenges: Barriers to effective implementation include concerns over data privacy, integration issues with legacy systems, and an ongoing skills shortage in the data science field.
- Future Trends: Emerging trends like real-time analytics and ethical AI are becoming vital to how data analytics is perceived and applied in the future.
These points not only underline the current landscape but also set the stage for future developments in the field of data analytics.
Implications for Future Research and Practice
Looking forward, there are several implications for future research and practice in data analytics:
- Need for Continuous Learning: As technology advances, professionals must partake in ongoing education and training to keep up with newer methods and tools.
- Focus on Ethics: The importance of ethical considerations in data analytics will continue to rise, necessitating frameworks that prioritize responsible data use.
- Integration with New Technologies: Research should explore how data analytics can be further integrated with other emerging technologies, such as the Internet of Things (IoT) or blockchain.
- Expansion of Applications: More extensive research is required to uncover new applications of data analytics in underexplored industries.
- Collaboration Across Disciplines: Interdisciplinary collaboration among fields such as computer science, statistics, and domain-specific knowledge will be crucial for innovative breakthroughs in data analytics.
In summary, the future of data analytics in industries holds substantial promise, but it will require collective efforts in research, ethical considerations, and technological integration to harness its full potential.