Decision Trees in Risk Management: A Comprehensive Exploration
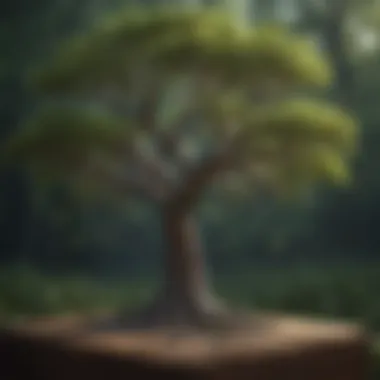
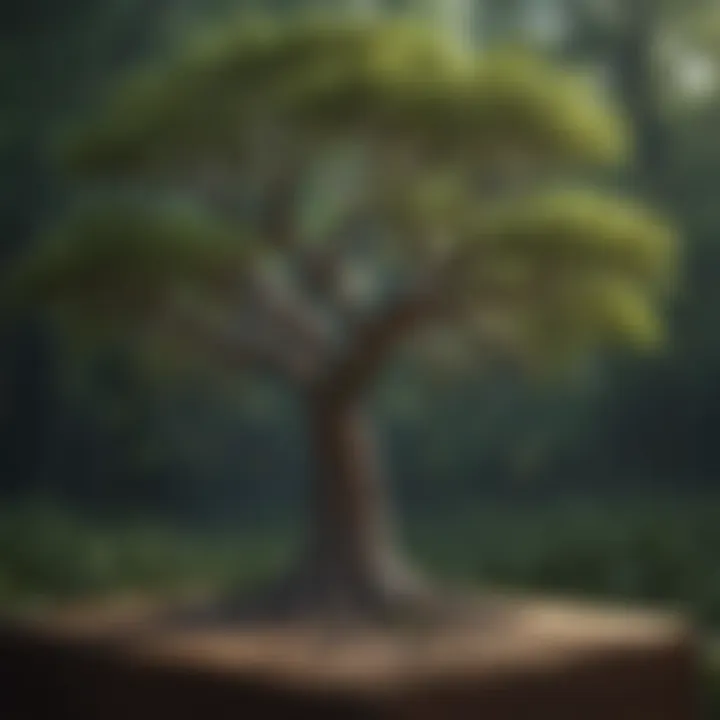
Intro
In recent years, the importance of structured decision-making frameworks has become increasingly undeniable. Among the various tools available, decision trees stand out due to their simplicity and effectiveness, especially in risk management. They can assist professionals in navigating complex scenarios where many variables are at play. This article will explore decision trees in detail, specifically focusing on their practical applications and relevance in the field of risk management.
Summary of Objectives
This section provides an overview of decision trees, outlining their essential components and functionalities. The objectives of this article include examining how decision trees enhance decision-making processes, the integration of these trees within various risk management frameworks, and highlighting their practical implications.
Importance of the Research
Understanding decision trees is vital for professionals involved in risk management. As organizations face increasing uncertainties, tools like decision trees offer a systematic way to evaluate potential outcomes. By employing decision trees, stakeholders can make more informed choices and mitigate risks effectively.
Results and Discussion
Presentation of Findings
Decision trees offer a graphical representation of choices and their possible consequences, which can be particularly useful in risk assessment. They consist of nodes, branches, and leaves that represent various outcomes resulting from decisions made. Key findings reveal that decision trees help clarify complex decisions by breaking them down into manageable parts.
- Enhanced Clarity: Decision trees simplify the decision-making process.
- Risk Visualization: They facilitate a visual understanding of risks and rewards.
- Scalability: Applicable to various scenarios in different domains.
Implications of Results
The use of decision trees in risk management has profound implications. Organizations can implement decision trees to foster collaboration among team members, enabling open discussions about potential risks. This collaborative approach often leads to more comprehensive risk assessments. Furthermore, decision trees can serve as a training tool for educating staff on the intricacies of decision-making under uncertainty.
"Effective risk management requires not just foresight but also robust frameworks to make sense of the complexities involved."
The limitations of decision trees, such as oversimplification and computational challenges with very complex data, must also be acknowledged. These factors require that decision trees be used thoughtfully alongside other risk assessment tools. In doing so, organizations can strike a balance between simplicity and depth in their risk management strategies.
The End
This article serves as a foundational exploration of decision trees in risk management. Through a balanced view of their advantages and possible pitfalls, stakeholders can leverage this tool effectively. The growing complexity of today’s risk landscape makes a clear understanding and application of decision trees necessary for professionals aiming to enhance their decision-making frameworks.
Understanding Risk Management
Risk management is a vital component in any strategic planning process. It serves as a systematic approach to identifying, assessing, and prioritizing risks, followed by coordinated efforts to minimize, monitor, and control the probability or impact of unfortunate events. In this article, we will explore the nuances of using decision trees in risk management. The use of decision trees contributes significantly to the structure and clarity needed for effective risk assessment.
Defining Risk Management
Risk management can be defined as the process that an organization employs to manage the potential adverse effects of uncertainty. It involves several steps, including risk identification, risk analysis, and risk mitigation. This process helps organizations understand potential threats to their objectives and plan accordingly. An effective risk management strategy not only reduces the chances of negative outcomes but also enhances decision-making.
Organizations often face various types of risks such as financial, operational, strategic, and compliance risks. Understanding these risks is essential for developing proactive measures. Decisions made using a formalized approach can lead to better allocation of resources, improved safety, and greater overall resilience.
The Importance of Risk Management in Organizations
Risk management is crucial for organizations as it underscores the significance of preparing for uncertainties that can impact performance and profitability. Here are a few key reasons that highlight its importance:
- Enhances Decision-Making: A well-defined risk management process empowers decision-makers with the necessary insights, allowing for informed choices.
- Resource Allocation: It helps in allocating resources more efficiently by identifying potential threats and prioritizing responses based on risk level.
- Reputation Management: Organizations that manage risks effectively can avoid reputational damage, as they are better prepared for various crises.
- Regulatory Compliance: Many industries have stringent regulatory requirements that necessitate robust risk management practices.
Ultimately, the integration of risk management into organizational culture enhances both operational and strategic decision-making. Effective risk management enables organizations to not only survive potential disruptions but also to seize opportunities that arise from uncertainty.
"Risk management is not just about avoiding loss; it is about making informed decisions with confidence."
Foreword to Decision Trees
In risk management, decision trees serve as a critical tool to aid in the decision-making process. They provide a systematic approach to assess potential outcomes based on various actions. Understanding the structure and function of decision trees is essential because it allows organizations to visualize complex decisions clearly. This section aims to illustrate the significance of decision trees, emphasizing their effectiveness in quantifying risks and guiding strategic choices.
What is a Decision Tree?
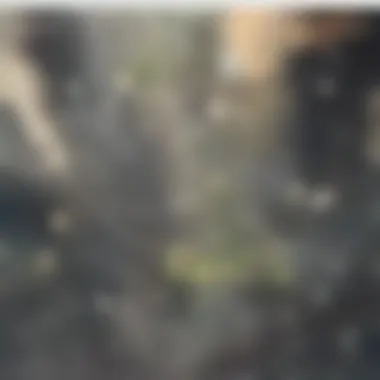
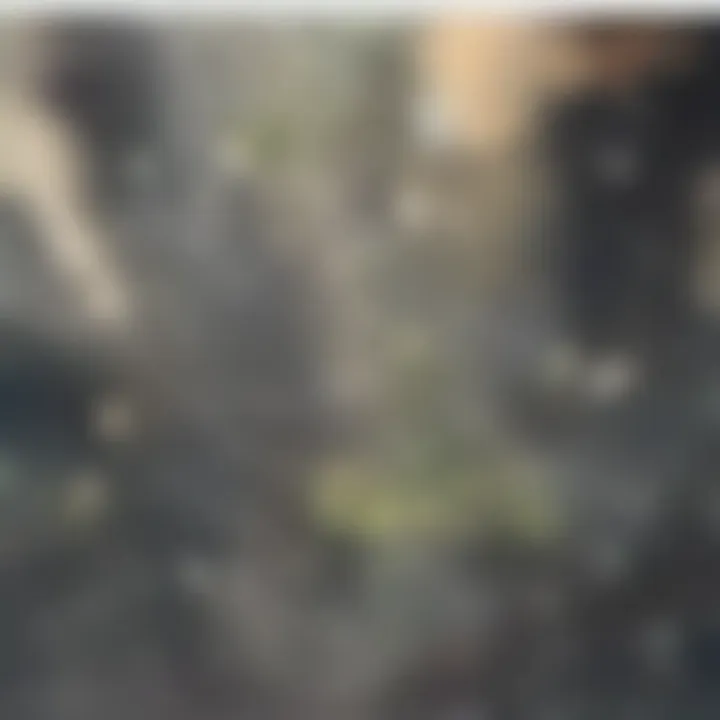
A decision tree is a graphical representation of choices and their possible consequences. It resembles a tree structure, where each branch signifies a decision, chance event, or outcome. By laying out alternatives, decision trees help clarify uncertainties, making them an invaluable tool in risk management. They become particularly useful when decisions need to reconcile multiple factors, creating a visual roadmap to navigate complex scenarios.
Components of a Decision Tree
The components of a decision tree include nodes, branches, and leaves. Understanding these elements is crucial, as they work together to form the structure that allows for detailed risk analysis. Each component contributes uniquely to the overall functionality of the decision tree.
Nodes
Nodes are the decision points within a decision tree. They represent choices that need to be made as part of a risk assessment. A key characteristic of nodes is that each one leads to different possible outcomes, depending on the choice made. This feature makes nodes a fundamental aspect of the tree, as they drive the exploration of various scenarios. The simplicity of nodes allows decision-makers to focus on key choices without being overwhelmed by complexity, thus enhancing clarity in risk evaluation.
Branches
Branches connect the nodes, illustrating the relationship between decisions and their consequences. This element is essential as it shows the flow of choices and outcomes. One of the primary benefits of branches is their ability to visually depict the different paths that decisions can take. They are particularly useful in constructive discussions about potential risks and alternatives. However, branches can also introduce complexity, especially in larger trees, making it vital to ensure that they are clearly defined and understandable to all involved parties.
Leaves
Leaves are the terminal points of a decision tree, representing final outcomes based on the decisions made. They encapsulate the results of various paths, allowing decision-makers to evaluate the ramifications of their choices. The unique feature of leaves is their role in summarizing outcomes succinctly, which aids in quick assessments of risk. Leaves provide a clear way to evaluate the implications of decisions, making them a popular choice for those seeking straightforward conclusions from complex scenarios.
Understanding the components of decision trees enhances the ability to manage risks effectively. Each element contributes to a clearer vision of potential outcomes and supports informed decision-making.
The Application of Decision Trees in Risk Management
The application of decision trees in risk management is an essential topic that warrants thorough examination. This systematic method assists in visualizing choices and their consequences, which can lead to more informed decisions. Decision trees offer a structured approach to dealing with uncertainty, allowing practitioners to illustrate complex processes in a way that is both straightforward and actionable.
Using decision trees, organizations can assess various risks and outline potential outcomes effectively. Their visual representation provides clarity that can help stakeholders understand the risks involved in a decision, fostering better communication and collaboration. Each choice leads to further options, which helps the decision-maker consider successive outcomes and their probabilities.
This section highlights specific benefits of employing decision trees as part of a risk management strategy. By facilitating clear decision-making, they are invaluable tools for organizations in various sectors. They not only help identify key risks but also clarify interactions among multiple variables, enhancing the overall risk assessment process.
Decision Trees for Risk Assessment
This subsection details how decision trees are crucial for risk assessment. When evaluating potential threats or hazards, decision trees allow analysts to systematically decompose complex scenarios into manageable parts. Each branch represents a decision point, and moving down the branches illustrates possible outcomes.
When constructing a decision tree for risk assessment, several aspects should be considered:
- Identifying Key Decisions: Start by determining what decision needs to be made. This involves gathering relevant data and assessing the context in which the decision is situated.
- Assessing Probability and Impact: Each branch of the tree represents a possible outcome. It is necessary to estimate the probability of each outcome and the potential impact it may have on the organization. This quantitative assessment helps in prioritizing risks effectively.
- Visualizing Consequences: One of the most significant advantages of decision trees is their ability to visually represent complex sequences of events. Stakeholders can quickly comprehend the potential consequences of different choices, allowing for more thoughtful considerations.
"The clarity provided by decision trees enables a more focused approach to identifying and mitigating risks."
By utilizing decision trees for risk assessment, organizations can derive better insights into potential risks, enhancing their ability to make proactive decisions based on data.
Scenario Analysis Using Decision Trees
Scenario analysis is another vital application of decision trees in risk management. This method allows organizations to evaluate various future scenarios and their potential effects on business operations. Decision trees make it possible to model different strategic paths based on varying assumptions and variables.
When conducting scenario analysis, one must keep the following elements in mind:
- Multiple Scenarios: Build trees that reflect different possible futures. This could include best-case, worst-case, and most-likely scenarios. Each scenario can show distinct paths, allowing decision-makers to visualize various outcomes.
- Correlating Risks and Outcomes: The relationships between different risks and their consequences can be complex. Decision trees help in establishing these correlations, making it easier to understand how one risk may impact another outcome.
- Dynamic Adjustments: As new information arises or circumstances change, decision trees can be updated accordingly. This flexibility is crucial for organizations needing to adapt their risk strategies swiftly.
Advantages of Decision Trees in Risk Management
The advantages of decision trees in risk management are critical to understanding their role in effective decision-making. Decision trees simplify complex decisions by providing a visual representation that delineates various options, outcomes, and their potential impacts. This clarity aids decision-makers in evaluating choices under uncertainty, facilitating not just understanding but actionable insights. In an era dominated by data and various risk factors, decision trees emerge as essential tools that offer several significant benefits.
Simplicity and Clarity
The simplicity of decision trees is one of their most appealing aspects. They transform complex scenarios into straightforward visual illustrations. Each decision point is represented as a node, branches show potential outcomes, and end points symbolize final results. This structure allows stakeholders to quickly comprehend the options available. For instance, consider a financial institution evaluating the risks of different investment strategies. By employing a decision tree, the institution can visualize the expected returns alongside associated risks, making it easier to choose the most viable option.
Moreover, decision trees do not require extensive statistical knowledge to interpret. They present information in an intuitive way that makes them accessible for practitioners at various levels. The visual format diminishes the cognitive load, enabling stakeholders to focus on the essence of the decision being made.
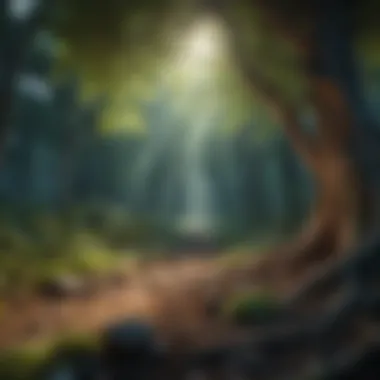
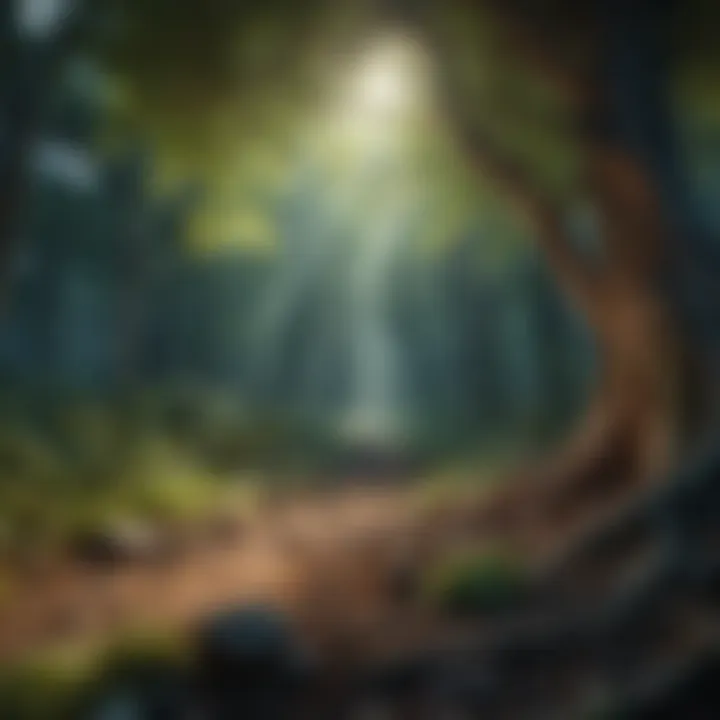
Facilitating Strategic Decision-Making
Strategic decision-making relies on the ability to evaluate various factors and potential scenarios effectively. Decision trees excel in providing a systematic approach to this evaluation. They allow decision-makers to analyze likely outcomes and the probabilities associated with each path. This capability is especially advantageous in fields such as finance, healthcare, and project management, where decision integrity is paramount.
Utilizing decision trees assists in prioritizing options based on risk and reward. For example, a project manager confronted with multiple project timelines can weigh the potential delays of each against their respective impacts. The ability to visualize consequences offers a comprehensive understanding of trade-offs involved.
Decision trees also highlight dependencies between decisions. This interconnected analysis encourages a more holistic view, ensuring that decisions contribute positively to overarching strategic goals.
Enhancing Communication of Risks
Effective communication is vital in risk management. Decision trees significantly enhance how risks are communicated among team members and stakeholders. By providing a graphical representation of risks and opportunities, they bridge the gap between technical jargon and actionable information.
This visualization fosters clarity when discussing complex risk scenarios. For example, stakeholders can collectively review the potential outcomes of a business decision, fostering more productive discussions. They can agree on the probability of different outcomes, aligning their understanding of potential risks.
In addition, decision trees serve as effective tools for documenting rationales behind decisions. This is particularly useful for future reviews or audits, allowing teams to analyze past decisions in the context of evolving landscapes. The structured format brings transparency, helping teams to explain the reasoning behind chosen strategies.
"The clarity provided by decision trees not only facilitates better decision-making but also supports collaborative discussions, ensuring all voices are heard."
Challenges and Limitations of Decision Trees
While decision trees are valuable tools in risk management, they are not without their challenges and limitations. Understanding these issues is crucial for professionals using decision trees for analysis and strategic decision-making. Each challenge can impact the reliability and effectiveness of decision-making processes, necessitating thoughtful consideration and strategies to mitigate their influence.
Overfitting Issues
One major challenge in the implementation of decision trees is overfitting. This occurs when a decision tree model captures noise rather than the underlying data distribution. In simple terms, a tree may become too complex, leading to predictions that are not generalizable to unseen data. When overfitting happens, it results in poor performance on new data.
A clear sign of overfitting is when a model performs well during training but poorly during validation.
Mitigating overfitting can be accomplished through techniques such as pruning, where branches of the tree that offer little predictive power are removed. This helps to simplify the model, improving its ability to generalize across different scenarios.
Handling of Continuous Variables
Continuous variables present another challenge for decision trees. They must be transformed into categorical data to create splits within the tree. This conversion can lead to loss of information if not handled carefully. Additionally, decision algorithms often need to make assumptions about how to categorize these continuous variables.
Improper handling can lead to suboptimal decision tree structures, affecting outcomes. One way to address this issue is by utilizing methods like binning, where continuous values are grouped into ranges. However, it is vital to maintain a balance, ensuring that the model retains relevant distinctions among data points.
Complexity of Large Trees
As decision trees grow larger, they become more complex, making interpretation difficult. Large trees with numerous branches can confuse stakeholders and dilute the clarity that decision trees strive to provide. Furthermore, complex trees can increase computational demands, complicating the analysis process.
It's important to recognize that while a detailed tree may seem advantageous, it often leads to confusing and less actionable insights. To counter this complexity, practitioners may choose to limit the maximum depth of trees or limit the number of branches to maintain a balance between detail and clarity.
In summary, while decision trees offer many benefits, professionals must remain aware of their challenges and limitations to harness their full potential in risk management. Understanding and addressing these issues will greatly enhance the robustness of decision-making processes.
Integrating Decision Trees with Other Risk Management Tools
In risk management, the integration of various tools enhances the depth and effectiveness of analysis. Decision trees serve as a foundational framework that can be effectively combined with other methodologies to provide richer insights. This section explores two significant integrations: Monte Carlo simulations and risk matrices. Each pairing presents unique advantages that strengthen decision-making processes in uncertain environments.
Combining with Monte Carlo Simulations
Monte Carlo simulations provide a mathematical framework for assessing risk through random sampling. When coupled with decision trees, this method becomes particularly powerful. Here’s how this combination works:
- Enhanced Probabilistic Analysis: Decision trees depict possible outcomes based on decisions made, while Monte Carlo simulations assess the probability of those outcomes occurring. The two approaches together allow a nuanced understanding of risk.
- Scenario Exploration: Decision trees outline various decision paths. Monte Carlo simulations help analyze these paths under different scenarios, providing data on how outcomes might fluctuate based on external factors. This synergy enables decision-makers to visualize a wider range of possibilities, thus enriching the risk management toolkit.
- Risk Quantification: By applying Monte Carlo simulations to decision trees, organizations can quantify risk more accurately. Not only do they see potential consequences, but they also get a statistical grasp of their likelihood, enabling more informed choices.
"The integration of Monte Carlo simulations with decision trees transforms abstract uncertainties into quantifiable risks, crucial for robust risk management."
Decision Trees and Risk Matrices
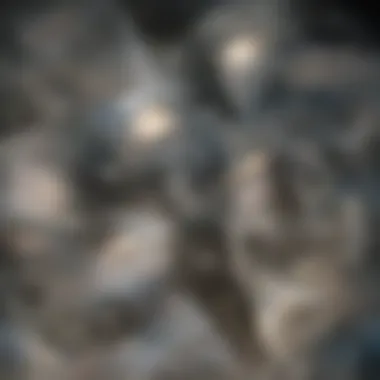
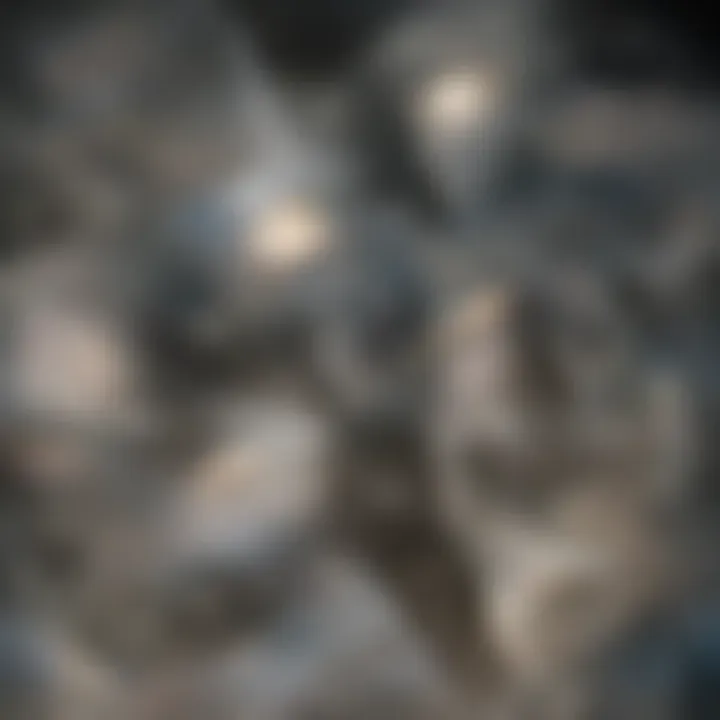
Combining decision trees with risk matrices adds a structured visualization element to risk assessments. Risk matrices are often used to classify the severity and likelihood of risks. Here are some key aspects of this integration:
- Risk Categorization: Risk matrices categorize risks based on their likelihood and impact. When a decision tree is overlaid on this matrix, it provides a visual representation of how decisions may shift risks from one category to another. Decision-makers can see not only potential risks but also manage them strategically.
- Facilitating Communication: Both tools are powerful in presentations. Risk matrices are intuitive. Decision trees add depth. Together, they help communicate complex risk scenarios effectively to stakeholders, ensuring that everyone involved understands the potential risks and decisions associated with them.
- Holistic Risk Assessment: Using decision trees in conjunction with risk matrices allows for a comprehensive view of risk. This dual approach ensures that all dimensions of risk are considered. Decision trees highlight processes; risk matrices focus on the outcomes.
In summary, the integration of decision trees with tools like Monte Carlo simulations and risk matrices offers enhanced analysis capabilities. It leads to improved risk assessments and informed decision-making, essential for effective risk management. The combination of these tools enables professionals to approach risks from multiple angles, ultimately fostering strategic thinking and a robust understanding of uncertainties.
Case Studies: Decision Trees in Action
The practical application of decision trees in real-world scenarios is essential to understand their value in risk management. Case studies provide tangible evidence of how decision trees function effectively within various sectors. They illustrate not only the process of decision-making but also the insights derived from data analysis. By examining real-life examples, readers can gain a nuanced perspective on the advantages and constraints of employing decision trees. Furthermore, these case studies highlight the adaptability of decision trees across different industries, reinforcing their role as a versatile tool in decision-making frameworks.
Case Study One: Financial Sector
In the financial sector, the use of decision trees can often guide investment decisions and risk assessments. A common scenario involves evaluating loan applications. Financial institutions employ decision trees to systematically assess the creditworthiness of applicants based on various factors such as income, credit history, and existing debts. The tree branches out into paths representing different potential outcomes, enabling analysts to visualize the probability of default.
Some benefits observed include:
- Clear Data Representation: Decision trees allow for straightforward visualization, making complex financial data accessible to stakeholders.
- Informed Decision-Making: By analyzing historical data patterns, financial analysts can make more calculated choices about lending.
- Risk Quantification: Decision trees help quantify risk, enabling institutions to establish appropriate interest rates based on an applicant's profile.
However, it is crucial to consider limitations, such as overfitting when trees become too complex, potentially leading to inaccurate predictions. Financial institutions must balance detail with clarity when crafting their decision trees.
Case Study Two: Healthcare Industry
In healthcare, decision trees serve as an essential tool for treatment planning and risk management. For instance, a hospital might utilize decision trees to determine the most suitable treatment for a patient based on symptoms and medical history. Each branch represents a different treatment path, and the outcome nodes show possible health scenarios following various interventions.
Key aspects include:
- Patient-Centric Decisions: Decision trees facilitate tailored healthcare plans, maximizing positive outcomes.
- Resource Allocation: By assessing various treatment options and their effectiveness, healthcare providers can allocate resources efficiently to higher-impact treatments.
- Enhanced Communication: Visual representation of risks and benefits aids discussions between healthcare professionals and patients, leading to informed consent decisions.
Nevertheless, challenges exist, such as the need for comprehensive data and the complexity of integrating these trees into existing decision-making processes. Overall, decision trees have shown to enhance clarity and efficiency, but practitioners must remain vigilant about the context-specific limitations.
Future Directions for Decision Trees in Risk Management
The field of risk management is evolving rapidly, especially with the integration of advanced technologies. Understanding the future directions for decision trees in this context is essential for professionals aiming to enhance their decision-making frameworks. As companies seek more robust methods to analyze risk, decision trees are likely to play a critical role in more sophisticated environments.
Advancements in Machine Learning
Machine learning (ML) has the potential to revolutionize many aspects of decision trees in risk management. Traditionally, decision trees required extensive manual input to define parameters and outcomes. However, ML algorithms can automatically analyze vast datasets to uncover patterns and correlations that may not be immediately visible to human analysts.
- Increased Efficiency: With the ability to process large amounts of data in real-time, organizations can utilize decision trees powered by ML to make quicker, more informed decisions.
- Adaptive Models: Machine learning enables continuous learning. As new data becomes available, decision trees can adjust their structure and predictions without human intervention. This adaptability improves the accuracy and relevance of risk assessments over time.
- Complex Decision-Making: Machine learning can define complex criteria and relationships that influence outcomes. Therefore, decision trees can handle multifactorial risk analyses, considering multiple variables that concurrently affect risk scenarios.
The Role of Big Data in Decision Making
Big data refers to large volumes of data that can be analyzed for insights, and its role in decision-making processes cannot be understated. Decision trees can leverage big data to enhance their effectiveness in risk management.
- Enhanced Data Sources: The integration of diverse datasets from social media, online transactions, and IoT devices adds depth to risk analysis. Big data allows decision trees to be more context-aware, providing a comprehensive view of potential risks.
- Predictive Analysis: With big data, decision trees improve their predictive power. They can analyze past behaviors and outcomes to forecast future risks more accurately. Businesses can plan better for uncertainties when they understand the predictive patterns underlying their data.
- Visualizing Complex Data: Big data technologies enable better visual representations of data within decision tree frameworks. Enhanced visualization helps stakeholders grasp intricate relationships and potential risks more effectively, thus improving communication and understanding.
Closure
The conclusion is a vital segment of this article. It synthesizes the insights discussed throughout and reinforces the significance of decision trees in risk management. This segment emphasizes the intricate balance between risk assessment and informed decision-making. The utilization of decision trees enhances understanding by visually structuring alternatives and outcomes, making complex data more comprehensible.
Summarizing Key Insights
In summary, decision trees serve as a valuable tool in risk management for several key reasons:
- Clarity: They provide a clear visual representation of choices, outcomes, and probabilities.
- Structured Analysis: Decision trees facilitate a systematic approach to evaluating risks, making it easier to quantify uncertainties.
- Application Flexibility: Their ability to integrate with other models widens their usability across various sectors.
- Scenario Planning: They support detailed scenario analysis, allowing professionals to strategize better.
These points elucidate how decision trees not only clarify risks but also serve as a foundation for strategic planning. Their structured nature aligns well with the dynamic needs of organizations.
Final Thoughts on Decision Trees in Risk Management
In closing, decision trees significantly enhance the landscape of risk management. Their design allows for thoughtful examination of potential outcomes against the backdrop of uncertainties. For professionals and organizations, using decision trees can drive more rational, data-driven choices.
The implications stretch across disciplines—from finance to healthcare—affirming that decision trees are not merely theoretical constructs but practical solutions addressing real-world challenges. Thus, as risk management evolves, so too should the methods we employ to navigate its complexities. Embracing tools like decision trees will undoubtedly fortify the decision-making frameworks that guide future organizational success.