Master Data Management: A Comprehensive Guide
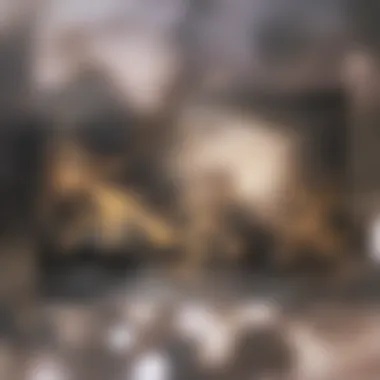
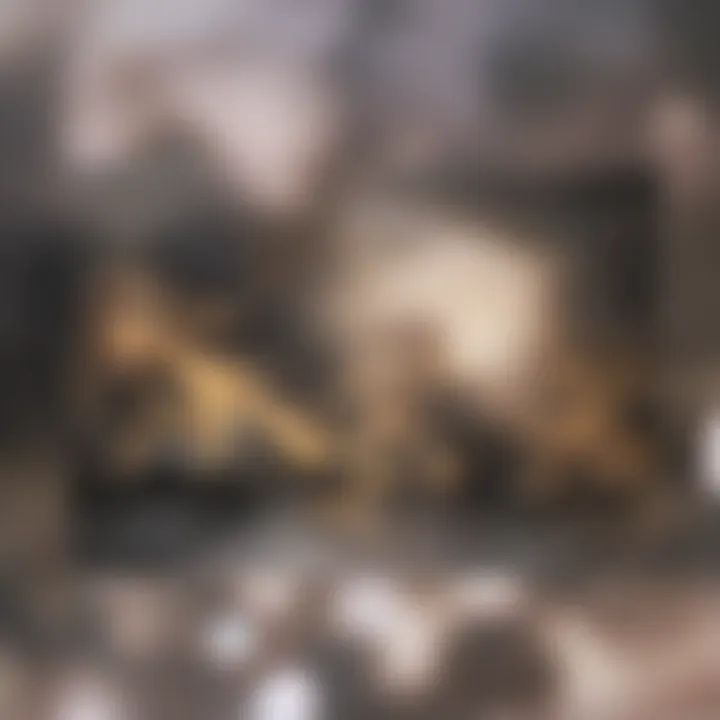
Intro
Master Data Management (MDM) is essential in todayβs data-driven business environment. It provides the framework for creating a single, accurate view of crucial data assets. This data may include customers, products, suppliers, and employees. Well-executed MDM processes help organizations manage their data consistently across multiple systems.
Understanding MDM begins with recognizing its fundamental principles. At its core, MDM involves the governance, quality, management, and integration of master data to ensure its accuracy and usability.
Summary of Objectives
The primary goal of this article is to outline the principles and best practices associated with MDM. We will explore effective strategies for implementation, dive into the role of technology, and examine the challenges faced by organizations in achieving optimal data management. By doing so, this comprehensive guide aims to provide valuable insights for both professionals and researchers in the field.
Importance of the Research
Researching MDM is important because organizations are generating vast amounts of data. The accuracy and consistency of this data are paramount for decision-making processes. Proper MDM practices can significantly improve operational efficiency, compliance, and customer satisfaction. Therefore, understanding MDM is not just beneficial; it is crucial for staying competitive and leveraging data effectively in todayβs environment.
Prelims to Master Data Management
The concept of Master Data Management (MDM) is pivotal for organizations aiming to maintain integrity in their data systems. In a landscape where data is a vital asset, understanding MDM can enhance how organizations manage and utilize their data. This section will dissect various elements integral to MDM, elucidate its significance, and pinpoint considerations that must be factored in to effectively implement it.
Definition and Importance
MDM refers to the policies and technologies utilized to ensure an organizationβs critical data assets are adequately maintained over time. Master data typically includes information about customers, products, employees, and suppliers. With consistent and centralized data management, MDM aims to eliminate data silos and inconsistencies.
The importance of MDM lies in its ability to foster data accuracy across the organization. It supports reliable decision-making and improves operational efficiency. In fact, many organizations face challenges stemming from inaccurate or fragmented data. By implementing a solid MDM strategy, companies can mitigate risks related to data discrepancies, which often lead to poor insights and misguided decisions.
Furthermore, regulatory requirements necessitate a strong framework for data governance. Adopting MDM makes it possible to not only meet compliance standards but also to enhance overall data quality. This leads to increased trustworthiness among stakeholders, which in turn fortifies customer relationships.
Key Components of
For MDM to be successful, it encompasses several key components:
- Data Modeling: Establishing the structure in which master data is represented and organized.
- Data Integration: Ensuring that data can be merged from various sources while remaining consistent and coherent.
- Data Governance: Creating policies and procedures that define who can access, modify, and delete master data.
- Data Quality Management: Regularly monitoring and cleansing data to maintain accuracy and trustworthiness.
- Workflow Management: Automating processes related to data handling, ensuring efficiency and compliance with established protocols.
These components build the foundation on which MDM operates. They facilitate the creation of a robust system capable of adapting to the evolving data landscape.
In summary, understanding the significance and components of MDM offers valuable insights. Companies that prioritize effective MDM not only achieve improved data quality but also gain a competitive edge. The investment in MDM strategies pays dividends by ensuring that reliable, high-quality data fuels the organizationβs decision-making processes.
Understanding Data Governance
Data governance is essential for the effective operation of Master Data Management. It encompasses the policies, procedures, and standards required to manage an organization's data assets. The significance of data governance in MDM cannot be overstated. Proper governance ensures that data is consistently defined, accurately maintained, and appropriately utilized across the enterprise.
Benefits of Data Governance
- Data Quality Improvement: Structured governance processes help to enhance data quality by setting standards and guidelines on data entry and maintenance.
- Compliance Assurance: Data governance frameworks can help organizations comply with legal and regulatory requirements, minimizing risks associated with data breaches.
- Ownership and Accountability: Governance assigns clear roles and responsibilities for data stewardship, fostering accountability and ownership among team members.
Hence, data governance acts as the backbone for successful MDM initiatives, promoting trust and effective decision-making based on solid data management principles.
Role of Data Governance in
The role of data governance within MDM is multifaceted. It provides structure, oversight, and accountability for all master data processes. Data governance ensures that data definitions and standards are uniformly applied across systems. Stakeholders in MDM rely on governance to clarify data ownership and to establish data stewards who maintain its integrity.
Moreover, data governance facilitates communication across departments regarding data usage and requirements. This is crucial because data often flows between different systems and divisions. Clear governance processes enable teams to understand and manage data's role within their operational contexts.
"Effective data governance is vital for mastering the art of data management."
Establishing Data Governance Frameworks
Building a robust data governance framework is critical for any organization looking to improve its MDM processes. A strong framework includes defined roles, responsibilities, and procedures for managing data. Here are some steps to consider in establishing a data governance framework:
- Assess Current Data Management Practices: Evaluate existing data processes to identify gaps and areas for improvement.
- Define Data Ownership: Assign ownership roles clearly to individuals or teams responsible for various datasets within the organization.
- Set Policies and Standards: Develop and document data management policies, including data quality standards and access control measures.
- Implement Training Programs: Invest time in training staff on data governance's importance and their specific roles to promote compliance and understanding.
- Use Technology Tools: Employ tools and software solutions that support data governance and facilitate processes like data cataloging and lineage tracking.
Continuous review of governance policies and procedures is vital. Frequent evaluations ensure relevance and efficiency as the organization evolves. By focusing on these areas, organizations can create a data governance framework that supports effective MDM and drives better business outcomes.
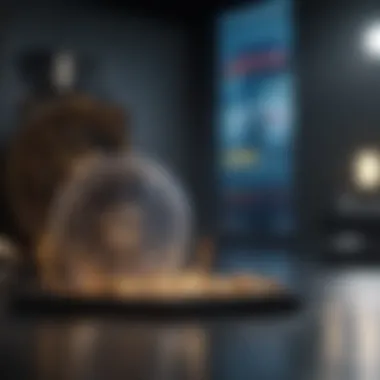
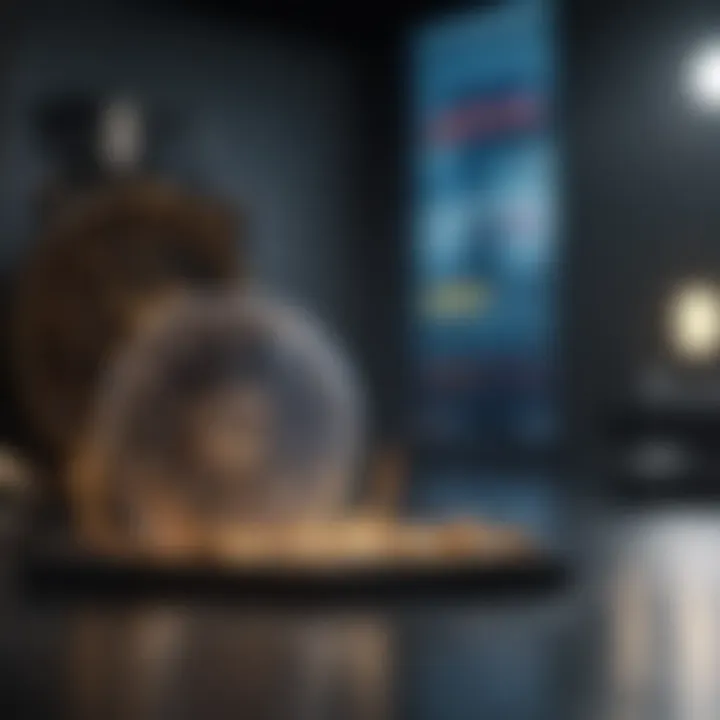
Implementation Strategies
MDM implementation strategies are crucial for ensuring that master data management is effectively integrated into an organization. Proper strategies can facilitate smoother transitions, reduce data inconsistency, and ultimately drive better business outcomes. Organizations need to recognize that MDM is not merely a technology issue but a comprehensive approach. It involves processes, governance, and culture, which are integral to its success. For many, grasping the right implementation strategies is the first step towards unlocking the full potential of their data.
Choosing the Right Model
When considering MDM models, organizations face a critical decision-making process. The choice of model significantly influences how data is managed and utilized across various departments. There are three primary models: the Registry model, the Consolidation model, and the Coexistence model. Each comes with its distinct advantages and considerations.
- Registry Model: This model creates a centralized registry of master data. It helps in identifying and matching data from various sources without any actual data consolidation. It is less intrusive and is often chosen by organizations that wish to retain existing systems intact.
- Consolidation Model: This model aggregates data from multiple sources. It provides a single view of the data by merging it in a central repository. This allows organizations to achieve higher quality data but requires more resources.
- Coexistence Model: This is a hybrid approach, combining elements from both the registry and consolidation models. It allows for flexibility, wherein organizations can choose to replicate or integrate data based upon specific business needs.
The right model varies based on factors such as organization size, complexity, and the current state of data infrastructure. Conducting a thorough assessment of these factors can guide organizations in making a well-informed decision that suits their unique requirements.
Steps for Successful Implementation
Implementing MDM requires a structured approach to realize its potential benefits. Here are key steps to consider:
- Define Objectives: Understand what the organization aims to achieve through MDM implementation. Clear objectives provide direction.
- Stakeholder Engagement: Involve all relevant stakeholders from the outset. When departments collaborate, the implementation process becomes smoother and more aligned with overall organizational goals.
- Data Assessment: Perform a comprehensive audit of current data. Identify data quality issues, such as duplicates or inaccuracies, that need addressing.
- Design the MDM Solution: Based on the chosen model, design the architecture. This includes considerations for software, hardware, and integration with existing systems.
- Develop Data Governance Policies: Establish clear policies concerning data ownership, quality, security, and compliance. This helps in maintaining data integrity over the long term.
- Execute the Plan: Begin the implementation process as per the designed architecture. Maintain continuous communication with stakeholders to address challenges as they arise.
- Monitor and Refine: Post-implementation, continuous monitoring is essential. Gather feedback on data usage and quality, allowing for ongoing improvements to processes and governance.
"Data is not just an asset; it must be managed in a way that maximizes its utility to the organizations."
By adhering to these steps, organizations can fortify their MDM strategies, enabling better decision-making, improved operational efficiency, and enhanced data quality across the board.
Technologies Supporting
The landscape of Master Data Management (MDM) is increasingly influenced by various technologies. These technologies are pivotal in ensuring that data is consistent, accurate, and relevant across the organization. They facilitate the integration, governance, and processing of data, which are crucial for a successful MDM framework. By leveraging modern technologies, organizations can enhance data management practices, support decision-making, and derive value from their data assets.
Software Solutions
MDM software solutions provide tools and platforms designed to centralize and manage master data effectively. These solutions are essential for organizations aiming to maintain data integrity and accuracy. Here are some key aspects of MDM software solutions:
- Centralized Repository: MDM software creates a single source of truth for master data, allowing organizations to manage, access, and enrich data from one location.
- Data Quality Management: Many MDM solutions include features for data cleansing, validation, and enrichment. This ensures that the data being used is of high quality.
- Workflow Automation: These solutions often have built-in workflow capabilities that streamline data management processes, reducing the time needed to update and maintain data.
- User Interfaces: Modern MDM software typically provides intuitive user interfaces, making it easier for users to interact with data and perform necessary functions.
- Real-time Data Updates: With the integration of real-time technologies, MDM solutions can keep data current and accurate by reflecting changes instantly.
In summary, MDM software solutions are vital for maintaining data integrity within organizations. They bring efficiency and accuracy to the data management process, which is essential for making informed business decisions.
Integration with Other Systems
Integration with other systems is another critical aspect of MDM technologies. An effective MDM strategy involves connecting various data sources and applications to ensure a seamless flow of information. Below are some components and benefits of integration:
- Connectivity: MDM systems should be able to connect with various platforms such as enterprise resource planning (ERP) systems, customer relationship management (CRM) systems, and other data sources.
- Data Synchronization: Integration facilitates synchronization of data across systems. This ensures that all departments are working with the same updated information, avoiding discrepancies that can lead to errors.
- Business Intelligence: When integrated with business intelligence tools, MDM systems can provide deeper insights and analyses. This allows organizations to make data-driven decisions based on comprehensive and accurate information.
- Scalability: An integrated MDM solution can grow with an organization. As more systems are added, the MDM framework can adapt and continue to provide a unified view of data.
Integrating MDM with existing systems not only enhances data accessibility but also improves overall organizational efficiency, which is increasingly vital in todayβs fast-paced business environment.
"Integration is not just a technical requirement but a strategic necessity for effective data management."
Effective MDM hinges on these technological solutions to ensure that data remains a valuable asset for organizations.
Benefits of Effective
Master Data Management (MDM) is vital for organizations that aim to optimize their data handling processes. By effectively managing master data, entities can experience numerous advantages that influence various aspects of operations. These benefits often translate into tangible outcomes and long-term success.
Enhanced Data Quality
Data quality directly impacts decisions made within an organization. With effective MDM, data is standardized, which reduces errors and discrepancies across various systems. Organizations can implement data validation processes that help to ensure accuracy.
- Standardization of data formats helps to eliminate confusion.
- Deduplication minimizes redundancy, leading to clearer datasets.
- Validation rules ensure that data entered meets specific criteria.
When data quality improves, operational efficiency rises. Employees spend less time correcting errors and more time utilizing accurate information for analysis and reporting. Therefore, enhanced data quality is not just about having correct data; it is also about fostering a more productive work environment.
Improved Decision Making
Good data leads to informed decisions. MDM provides a unified view of data across various departments. This consolidation empowers managers and executives to base their strategies on comprehensive insights rather than fragmented information.

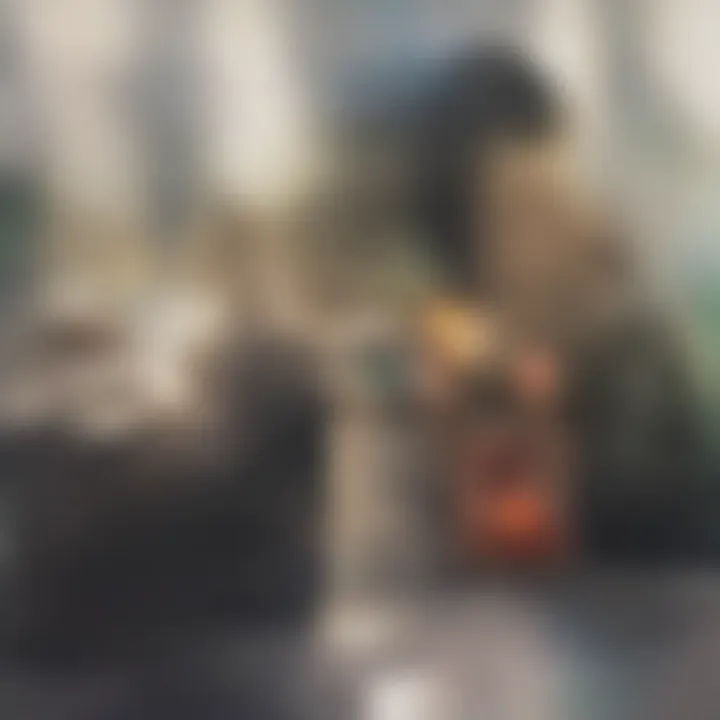
- A holistic view of customer data can improve targeting for marketing campaigns.
- Financial forecasts become more reliable with accurate data entries from multiple sources.
- Operational decisions can be streamlined using real-time reporting capabilities.
In essence, improved decision making is a result of data arriving at the right time, in the right form, to support strategic planning.
Regulatory Compliance
Regulatory compliance is crucial in todayβs business landscape. Institutions must adhere to various laws and standards that govern data usage. Effective MDM helps organizations maintain compliance by ensuring observance of data regulations, such as GDPR or HIPAA, depending on their industry.
- Data lineage tracking assures that organizations know where their data originates and how it is used.
- Regular audits of master data ensure compliance with legal requirements.
- A well-documented data management process can serve as evidence of compliance during regulatory reviews.
MDM not only aids in staying compliant but also protects reputations and minimizes the risk of legal challenges.
"Effective management of master data enhances overall business efficiency and mitigates risks associated with poor data usage."
Challenges in
Master Data Management (MDM) is essential for maintaining data integrity across an organization. However, implementing it is not without difficulties. Understanding the challenges in MDM can help organizations navigate these obstacles more effectively. This section emphasizes specific elements related to these challenges, their impacts, and considerations necessary for successful MDM.
Data Silos and Integration Issues
Data silos occur when information is isolated within different departments or systems, making it difficult to obtain a unified view of data. Integration issues arise when various data sources cannot communicate effectively, complicating data management. The existence of silos can significantly hinder the objectives of MDM, which emphasizes a single source of truth.
Data must flow freely among different systems to maintain accuracy and consistency. Failure to integrate leads to duplicate data, outdated information, and discrepancies. Such issues can affect decision-making processes negatively. Therefore, organizations should prioritize strategies for breaking down these silos and enhancing integration.
- Establish Communication Protocols: Develop standard procedures for data sharing across departments.
- Utilize Middleware Solutions: Middleware can bridge gaps between incompatible software, ensuring seamless data transfer.
- Promote a Unified Data Strategy: Align departments under a common data management strategy to encourage collaboration.
"The effective management of data silos is crucial for fostering a cohesive and comprehensive view of information within an enterprise."
Change Management Concerns
Change management is another significant challenge in MDM. As organizations evolve and adopt new technologies, aligning people, processes, and data can become complex. Employees may resist changes due to uncertainty or lack of understanding of new systems. This resistance can derail MDM initiatives, leading to incomplete implementation and insufficient user adoption.
To address change management concerns, organizations should embrace a structured change management process. This includes:
- Training and Education: Provide thorough training programs to familiarize employees with new tools and practices.
- Stakeholder Engagement: Involve stakeholders in the decision-making process to foster buy-in and address concerns proactively.
- Continuous Feedback: Implement mechanisms for continuous feedback to assess employee sentiments and adapt the change process accordingly.
By prioritizing change management alongside technical implementation, organizations can facilitate smoother transitions and greater acceptance of MDM practices.
Best Practices in
Master Data Management (MDM) requires the adoption of best practices to ensure success and sustainability. Implementing effective MDM is not limited to technology alone. It is a holistic approach that includes people, processes, and governance. Establishing best practices can significantly enhance data consistency, improve decision-making, and foster accountability across an organization.
Creating a Data-Centric Culture
Creating a data-centric culture is crucial for the success of MDM. This culture promotes the idea that data is a strategic asset, and every employee plays a role in managing it. It starts by educating all levels of the organization about the importance of high-quality data. When employees understand how their inputs affect overall data integrity, they are more likely to take ownership.
Key elements to consider:
- Training and Awareness: Regular training sessions help employees recognize the value of accurate data.
- Collaboration Between Departments: Encourage communication among teams to share insights and best practices.
- Data Quality Champions: Appoint individuals responsible for enforcing data governance policies.
Adopting such practices leads to enhanced data quality, making it easier to derive insights and make strategic decisions. It creates an environment where everyone is on board with the importance of maintaining data integrity.
Continuous Monitoring and Improvement
Continuous monitoring of MDM processes is vital for ensuring that data remains accurate and useful. Organizations should not view MDM as a one-time project but rather as an ongoing process that evolves over time.
Consider these strategies for effective monitoring:
- Regular Audits: Conduct audits to identify data inconsistencies and rectify issues promptly.
- Feedback Mechanism: Establish channels for employees to report data quality issues or improvement suggestions.
- Key Performance Indicators (KPIs): Set and track KPIs related to data quality, such as accuracy rates or the frequency of data errors.
By focusing on continuous improvement, organizations can adapt to changing business needs and address emerging data challenges. This flexibility ensures that the MDM framework evolves in step with the organization, maintaining relevance and effectiveness in data management.
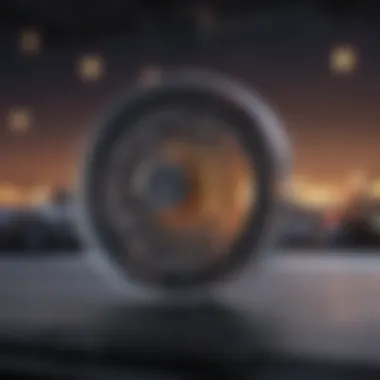
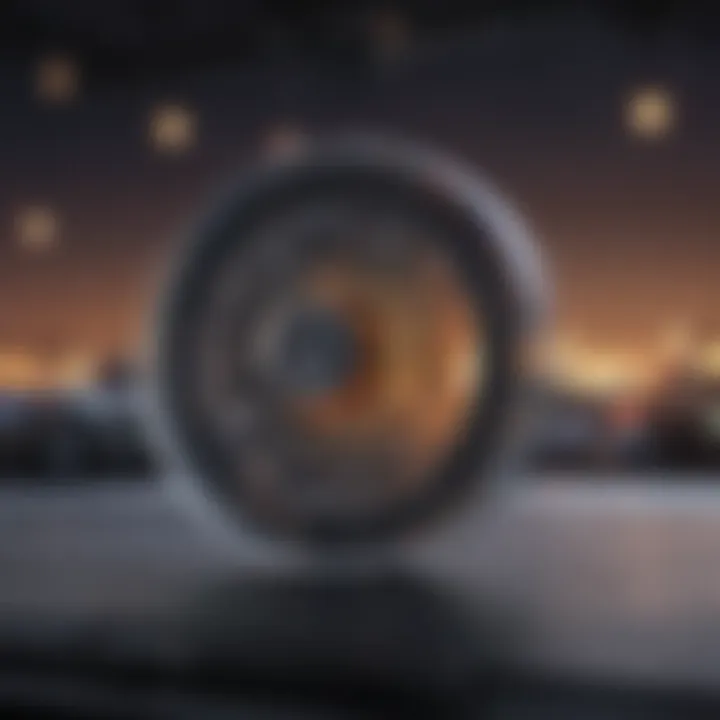
Future Trends in Master Data Management
Master Data Management (MDM) is constantly evolving. As technology advances, businesses must adapt to maintain competitive advantage. Future trends in MDM are pivotal for organizations seeking to enhance their data governance, accuracy, and accountability. Understanding these trends is more than helpful; it is necessary for future-proofing data management practices.
Adoption of Artificial Intelligence
Artificial Intelligence (AI) is reshaping many industries. It significantly impacts MDM by automating processes and improving data quality. AI can analyze vast amounts of data and identify patterns more efficiently than humans. This can lead to better decision-making, reduced errors, and enhanced productivity.
Some key benefits of AI in MDM include:
- Data Cleansing: AI algorithms can identify and rectify inconsistencies in datasets, ensuring better data quality.
- Predictive Analytics: Organizations can leverage AI to anticipate trends and behaviors based on historical data, enabling proactive strategies.
- Enhanced Data Matching: AI can improve the accuracy of matching records across different systems, thus reducing data duplication.
However, implementing AI requires thoughtful considerations, such as data privacy and the limitations of algorithms. Organizations must balance the need for innovation with ethical considerations.
The Role of Cloud Computing
Cloud computing is revolutionizing how organizations manage their data. It offers scalable infrastructure and resources without requiring significant upfront investments. For MDM, cloud solutions provide benefits that traditional systems may lack.
Historically, MDM solutions were dependent on on-premises setups. Now, cloud-based MDM systems facilitate:
- Scalability: Organizations can easily scale their data resources as needed, ensuring performance aligns with business growth.
- Accessibility: Cloud solutions allow access to master data from anywhere, promoting collaboration and efficiency.
- Cost Efficiency: By reducing hardware costs, cloud computing makes MDM solutions more accessible to smaller organizations.
Moreover, cloud platforms enhance integrations with other systems, ensuring that data flows seamlessly across applications.
"Cloud computing enables organizations to manage master data in a flexible, cost-effective, and scalable way."
Case Studies in
Master Data Management (MDM) case studies play a vital role in illustrating the practical application of MDM principles across different industries. They provide tangible examples of how organizations navigate the complexities of data management, revealing successes and failures alike. Analyzing these case studies offers insights into effective strategies, challenges overcome, and lessons learned.
Studying these cases enables organizations to better understand the landscape of MDM solutions. It helps in identifying what works and what does not in real-world settings, thus aiding in informed decision-making for other enterprises. Moreover, case studies can showcase advancements in technology and methodologies, highlighting innovative solutions that solve common data management issues.
"Case studies in MDM not only highlight the importance of data accuracy but also illuminate the significant impact on business performance and efficiency."
Industries Successfully Implementing
Several industries have successfully adopted MDM to streamline processes and ensure data integrity. Each industry has unique requirements and challenges, yet they all share the goal of mastering data to enhance decision-making capabilities.
- Healthcare: In this sector, MDM ensures accurate patient records, improving patient care and operational efficiency. Healthcare organizations like Mayo Clinic have implemented MDM to unify disparate systems, enabling seamless access to patient data across platforms.
- Financial Services: Banks and financial institutions, such as JPMorgan Chase, utilize MDM for compliance and risk management. In managing customer and transaction data, they achieve a single view of their clients, enhancing regulatory adherence and decision-making quality.
- Retail: Major retailers like Walmart use MDM to maintain accurate inventories and customer records. This optimizes supply chain management, resulting in better stock handling and improved customer satisfaction through personalized marketing.
- Manufacturing: Companies such as Siemens implement MDM to manage vast amounts of material and supplier data. Doing so aids in operational consistency and enhances product quality through better data management.
Lessons Learned from Failures
Examining failures in MDM provides important lessons that can guide future implementations.
- Lack of Executive Buy-in: Often, MDM initiatives fail due to insufficient support from top management. Without executive sponsorship, projects may lack necessary resources and strategic direction.
- Inadequate Change Management: Change resistance among staff can derail MDM efforts. Organizations have learned that a clear change management strategy is crucial for successful adoption.
- Underestimating Data Quality Issues: Companies sometimes overlook existing data problems during MDM implementation. Ensuring data quality before embarking on MDM initiatives is essential. Lack of data cleansing can lead to sour results.
- Failure to Align MDM with Business Goals: MDM must align with overall business strategies. Projects that do not consider the larger organizational objectives are prone to becoming irrelevant or ineffective.
Ending
The conclusion of an article on Master Data Management (MDM) is not merely a summary; rather, it serves as a pivotal point that distills the essence of the discussions and insights presented throughout. This section is crucial as it reinforces the importance of mastering data management in todayβs dynamic organizational environments.
Summarizing Key Insights
In the journey through the various aspects of MDM, several key insights emerge:
- Consistency and Accuracy: MDM provides a framework that ensures the consistency and accuracy of data across departments. With a solid master data governance process, organizations can minimize the risk of data discrepancies and enhance trust amongst users.
- Enhanced Decision-Making: Quality data supported by MDM allows for improved decision-making. Reliable master data means that strategic decisions are made based on valid insights rather than flawed assumptions.
- Operational Efficiency: Integrating MDM practices leads to operational efficiencies. By eliminating data silos, organizations can reduce redundancy and improve processes, which in turn supports more agile and responsive business operations.
"Effective MDM not only safeguards data but also transforms it into a strategic asset that drives value across the organization."
- Regulatory Compliance: With stringent regulations surrounding data, effective MDM helps organizations maintain compliance, thus avoiding penalties and enhancing their reputation.
These insights underline the critical need for structured and well-implemented data governance frameworks that can support enduring success in MDM applications.
The Future of Data Management
The landscape of data management is rapidly evolving. As we look ahead, several trends will shape the future of MDM:
- Artificial Intelligence: The integration of AI technologies will revolutionize MDM, enabling advanced analytics, automated data cleansing, and predictive insights. As AI continues to mature, its use in MDM will become indispensable for organizations seeking a competitive edge.
- Cloud Computing: The shift towards cloud-based solutions is transforming how companies approach MDM. Cloud platforms facilitate accessibility, offering organizations scalable solutions that reduce overhead costs and improve collaboration.
- Data Privacy and Security: Increased awareness around data privacy will necessitate more robust governance practices. Organizations will need to incorporate modern security measures to protect sensitive data while maintaining compliance with evolving regulations.
- Real-Time Data Management: The demand for real-time insights is rising. As businesses strive for agility, MDM systems will need to adapt to support the real-time processing of data, ensuring timely access to accurate information.