Mastering Business Statistics for Effective Decision Making
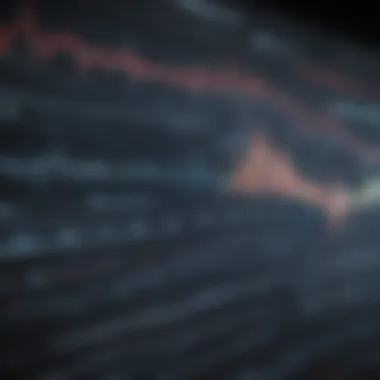
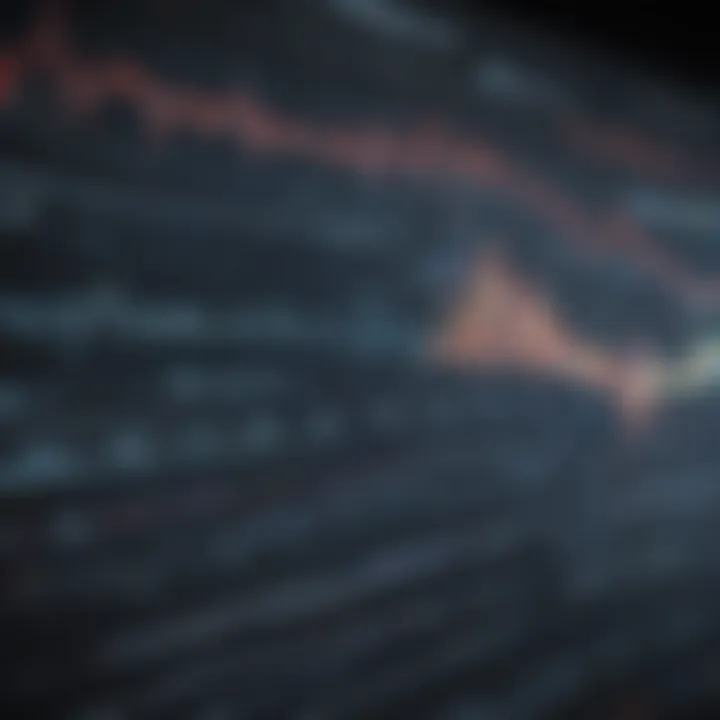
Intro
In today's fast-paced world, business statistics is a crucial discipline. To navigate the complex landscape of commerce, understanding statistics is not merely an added advantage β it's often a necessity. The way we collect, analyze, and interpret data significantly influences decision-making and strategizing in organizations large and small.
Business statistics can be seen as the heartbeat of informed decision-making. Without it, companies are like ships without a compass, drifting aimlessly in an unpredictable ocean. Statistics allows businesses to convert raw data into actionable insights, helping them to refine strategies, mitigate risks, and seize opportunities.
Why We Focus on Business Statistics
Engaging with business statistics transcends mere number crunching. It encompasses a series of methodologies aimed at ensuring data integrity and accuracy while providing valid analysis for what the future could hold. It's all about harnessing the power of data.
To better appreciate its relevance, consider the various realms where statistical methods come into play:
- Market research: Identifies potential customers and needs.
- Quality control: Ensures products meet certain standards before hitting the shelves.
- Financial forecasting: Predicts future revenues and expenses based on historical data.
But the importance of statistics doesn't stop there; it serves as a bridge between theory and practice. By mastering statistical tools, stakeholders can make evidence-based decisions, which can be the difference between thriving and merely surviving in todayβs competitive marketplaces.
Statistics, when executed correctly, articulates a narrative within the numbers. It delivers a comprehensive analysis of performance evaluation, facilitating strategic planning that can propel organizations to heights they never thought possible.
Prelims to Business Statistics
Business statistics serve as a cornerstone for sound decision-making in a myriad of industries. By analyzing data, organizations can uncover patterns, make predictions, and strategize effectively. In todayβs rapidly evolving marketplace, the ability to interpret statistical information is more than just advantageous; it's essential. Statistical insights empower firms to gauge market trends, customer preferences, and operational efficiencies, thus providing a competitive edge. Understanding business statistics is akin to having a roadmapβa clear illustration of where to direct resources and efforts for maximum impact.
Defining Business Statistics
At its core, business statistics is the science of collecting, analyzing, presenting, and interpreting data in a business context. It encompasses a wide range of methodologies and techniques that facilitate informed decision-making. Dive into the main components:
- Data Collection: Gathering relevant information through various methods like surveys, experiments, and observations.
- Data Analysis: Employing statistical tools to discern meaningful insights from the raw data, revealing trends or correlations that might not be immediately evident.
- Data Presentation: Using visualsβcharts, graphs, tablesβto effectively communicate findings to stakeholders.
- Data Interpretation: Making sense of the analyzed data to guide strategy, with a focus on applications that drive business objectives.
In this way, business statistics transforms numbers into narratives that inform company strategies and operational practices.
Importance in Business Environments
The relevance of business statistics in organizational frameworks cannot be overstated. Here are key reasons why it matters:
- Informed Decision Making: Business statistics provides data-driven insights, allowing for more accurate forecasting and evaluation of risks associated with decisions.
- Improved Efficiency: By analyzing operational data, companies can identify inefficiencies and areas for improvement, streamline processes, and ultimately reduce costs.
- Enhanced Customer Understanding: Statistics facilitate a deeper comprehension of customer behaviors and preferences, leading to better-targeted marketing strategies.
"Statistics is the language of business. Without understanding it, youβre merely wandering in the dark."
On the whole, the tools of business statistics enable organizations to adapt more swiftly to changes in the marketplace, making them not just reactive, but proactive as well. This ensures sustained growth and relevance in an environment characterized by constant change.
Data Collection Methods
Data collection methods are crucial in the realm of business statistics, setting the stage for all subsequent analyses. This foundational step ensures that the information harnessed is not only relevant but also accurate. Identifying the right data collection approach can significantly impact the effectiveness of research outcomes. Whether businesses seek to gauge customer satisfaction, assess market conditions, or evaluate operational efficiencies, the methods chosen will dictate the quality of insights derived.
Quantitative vs. Qualitative Data
When discussing data collection, two primary categories emerge: quantitative and qualitative data. Quantitative data refers to numerical information that can be measured and quantified. Examples include sales figures, employee headcounts, and customer ratings on a scale from one to ten. This form of data usually enables straightforward interpretation, useful for statistical analysis and hypothesis testing.
On the flip side, qualitative data encompasses descriptive insights that capture the nuanced opinions and experiences of individuals. This might include open-ended survey responses or interview transcripts. While qualitative data provides depth and context, it is inherently more subjective. Both forms of data serve distinct purposes. Quantitative data can reveal patterns and trends, while qualitative data often sheds light on motivations, attitudes, and underlying reasons behind the numbers.
"The interplay between quantitative and qualitative data can deliver a fuller picture of the business landscape."
Surveys and Questionnaires
Surveys and questionnaires stand out as powerful tools in data collection. They afford businesses the opportunity to gather information directly from a target audience, be it employees, customers, or stakeholders. A well-crafted survey can yield high-quality quantitative data, especially when employing closed-ended questions that allow for statistical analyses.
However, simple surveys can also incorporate open-ended questions to tap into qualitative insights. For instance, a restaurant might ask customers to rate their dining experience on a scale of one to ten, and provide a free-form space to share any additional comments. This dual approach offers a richer dataset, allowing for analysis of numerical trends alongside personal feedback.
When designing surveys, consider the following elements:
- Clarity of Questions: Ensure questions are straightforward and unambiguous.
- Target Audience: Tailor the survey to suit the demographic being studied.
- Length: Keep surveys concise to avoid participant fatigue.
Observational Studies
Observational studies represent another method of data collection. This approach involves systematically watching subjects in their natural environment without manipulation or intervention. It is particularly fruitful in environments where behaviors or actions must be understood in real time.
For example, a company might observe customer interactions within a store to identify preferences or issues affecting their shopping experience. This technique often captures data that survey responses or interviews might overlook, especially when it comes to spontaneous behaviors or unfiltered reactions. Observational data can be seen as primary evidence of real-world behaviors, but it does present its own set of challenges, such as observer bias and the difficulty of replicability.
Statistical Analysis Techniques
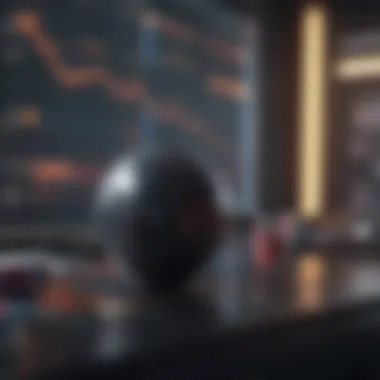
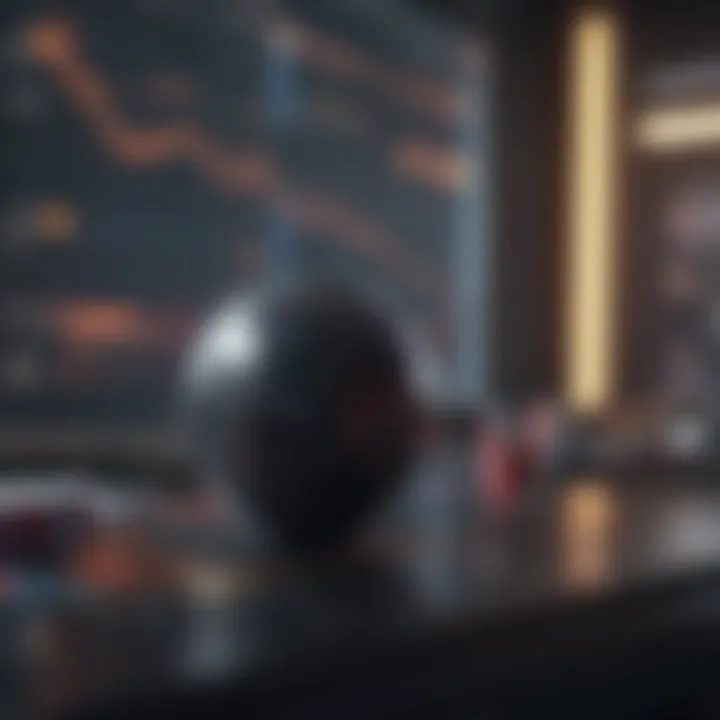
Statistical analysis techniques are the backbone of understanding and interpreting data in the realm of business. They provide the tools necessary to convert raw numbers into actionable insights, giving businesses the edge in decision-making processes. Understanding these methods broadens perspectives, helping professionals gauge performance, forecast trends, and even identify potential pitfalls.
The significance of statistical techniques cannot be overstated. They help to increase precision when estimating the outcomes of various business scenarios. Not only do these techniques shed light on current performance metrics, but they also prepare organizations for future challenges, serving as a guide based on historical data.
Descriptive Statistics
Descriptive statistics are often the first step in any data analysis plan. They help summarize and present data in a meaningful way.
Measures of Central Tendency
Measures of Central Tendency, which include the mean, median, and mode, play a crucial role in summarizing the center point of a data set. One distinctive feature of these measures is that they provide a singular value that reflects a typical data point, making it easier for businesses to gauge performance. For instance, knowing the average sales figures can help a business assess how they stack up against competitors.
This measure is popular because it gives a simple view of data, aiding quick and effective decision-making. However, while central tendency points toward common behavior, it can sometimes obscure significant variations within the data. For example, relying solely on the average can lead to misguided assumptions if the data has notable outliers.
Measures of Variation
Measures of Variation, including range, variance, and standard deviation, give insight into how spread out the data points are around the central tendency. This aspect allows businesses to understand not just the typical outcome but how consistent that outcome is, which is vital for risk assessment.
The unique feature of these measures lies in their ability to highlight diversity in data. For instance, two businesses might report similar average sales, but one may fluctuate greatly while the other remains stable. Understanding this variability can significantly impact strategic plans. However, while measuring variation provides powerful insights, it requires careful interpretation to avoid drawing hasty conclusions.
Inferential Statistics
Inferential statistics take data analysis a step further by making predictions and generalizations about a population based on a sample. This branch is vital for testing theories and making informed decisions in uncertain environments.
Confidence Intervals
Confidence Intervals offer a range within which we can expect a population parameter to lie, based on sample data. This characteristic is valuable for businesses as it allows them to make estimates with a degree of certainty. For instance, a company can use confidence intervals to project future sales numbers with attached levels of certainty.
What makes confidence intervals attractive is their ability to encapsulate uncertainty while still providing actionable data. A common challenge, though, is ensuring the correct sample size and methods; otherwise, the intervals may mislead.
Hypothesis Testing
Hypothesis Testing is a statistical method used to determine if there is enough evidence to support a particular claim about a population. It is fundamental for business scenarios where confirming suspicion or disproving myths is crucial. A characteristic of hypothesis testing is that it evaluates two opposite hypotheses: the null hypothesis and the alternative hypothesis.
This technique is notably beneficial for making data-driven decisions. However, the limits of hypothesis testing come into play when the data is not representative, which can lead to Type I or Type II errors.
Regression Analysis
Regression Analysis is a statistical technique used to understand relationships between variables. Itβs a robust method that helps predict outcomes based on independent variables, making it especially useful in strategic planning.
Simple Linear Regression
Simple Linear Regression involves finding a linear relationship between two variables, which can help businesses foresee the outcome of one variable based on the changes in another. Its key characteristic is the straightforward formula that defines this relationship, making it accessible for most users. For example, if a company wants to know how advertising spend impacts sales, this method offers a clean answer.
However, while it does provide clarity, simple linear regression is limited by its focus on only two variables, which often doesn't capture complexities of real-world scenarios.
Multiple Regression
Multiple Regression expands on the idea of its simpler counterpart by examining the relationships between one dependent variable and multiple independent variables. This method gives deeper insights into how various factors collectively impact outcomes, allowing businesses to strategize more effectively.
The unique advantage is its capacity to consider interdependencies among numerous factors. For instance, understanding the influence of price, quality, and marketing on product sales can lead to comprehensive strategies. Nonetheless, interpreting multiple regression requires careful consideration of multicollinearity, where the independent variables may be correlated, complicating the analysis.
Data Interpretation and Presentation
Data interpretation and presentation serve as the linchpin in the realm of business statistics. Without these crucial elements, the vast amounts of data collected and analyzed remain just that: mere numbers and figures, lacking the context and clarity needed for informed decision-making. This section unpacks the essentials of interpreting data meaningfully and presenting it effectively, allowing stakeholders to absorb insights that drive strategic initiatives.
Visualizing Data with Graphs
Graphs are often the bridge between raw data and compelling insight. In simple terms, they transform complex datasets into visual narratives that audiences can grasp at a glance. When executed well, a graph can convey trends, patterns, and outliers far more powerfully than tables overflowing with figures.
Key types of visualizations include
- Bar graphs: Ideal for comparing categories.
- Line charts: Excellent for displaying data trends over time.
- Pie charts: Effective for showing proportions within a whole.
When designing graphics, it is crucial to consider clarity and accuracy. Avoid clutter. A busy graph can muddle the message, leading to confusion rather than enlightenment. Labels and legends should be straightforward, and color choices must enhance understanding without overwhelming the viewer.
"Good data visualization is like good architecture. Itβs not just about aesthetics; itβs about function, clarity and the narrative it conveys."
Moreover, incorporating interactive elements in presentations can offer audiences deeper dives into the data. This fosters engagement and allows users to manipulate the data for personalized insights. Tools like Tableau or even Excel offer capabilities to create dynamic and engaging representations that keep stakeholders involved.
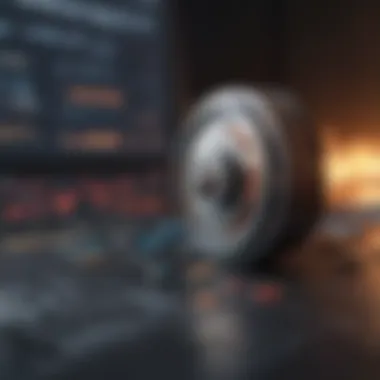
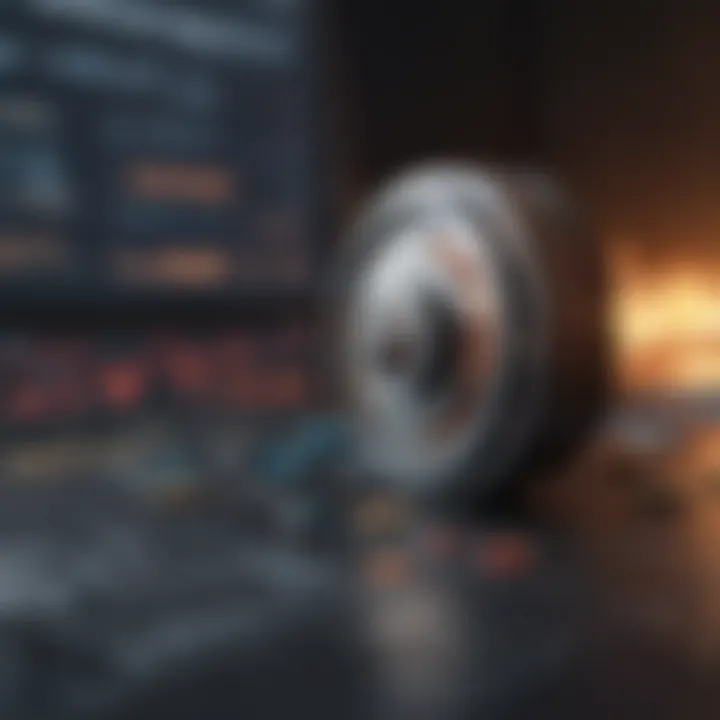
Crafting Effective Reports
Crafting a report involves storytelling β and like any good story, it has a beginning, middle, and end. A well-structured report should present findings in a logical flow, guiding the reader from the introduction of the problem or question, through the methodology, results, and finally to the conclusions and recommendations. Each section must work together seamlessly.
Consider the following components in your reports:
- Executive Summary: A concise overview to grab attention.
- Introduction: Set the stage; clearly define the objectives of the study or analysis.
- Methodology: Describe how the data was collected and analyzed. This section builds credibility.
- Results and Discussion: Present findings in a clear, straightforward manner, supported by visual aids as discussed in the previous section.
- Conclusion and Recommendations: Where the rubber meets the road. Summarize the insights and suggest actionable steps based on the data.
In todayβs data-driven world, reports also need to be accessible. Make them engaging by using a clear, informal writing style that invites rather than intimidates. Avoid jargon; if terms are necessary, include a glossary for clarity.
The Role of Software in Business Statistics
In the ever-evolving landscape of business, the role of software in the realm of statistics cannot be understated. As organizations strive for efficiency and precision, these tools become the backbone of data manipulation and analysis. They facilitate the ability to handle large datasets, automate repetitive tasks, and execute complex calculations with ease. For businesses aiming to make data-driven decisions, harnessing the capabilities of statistical software is not just advantageous; itβs essential.
Software simplifies the statistical process by providing robust frameworks for data analysis. Whether a company is conducting market research or developing forecasts, the right statistical tools can make the journey smoother and more insightful.
Statistical Software Overview
SPSS
When it comes to statistical software, SPSS stands tall. Itβs known for its user-friendly interface, which makes statistical analysis accessible even for those without a profound statistical background. A standout feature is its extensive library of statistical tests, allowing users to quickly gather insights from their data.
SPSS shines particularly in social sciences but is applicable in various fields. Its ability to handle complex data manipulations, like MANOVA and factor analysis, makes it a popular choice for many researchers. However, SPSS can be pricey, which might pose challenges for smaller businesses or students.
R
R represents a powerful open-source programming language specifically designed for statistical computing and graphics. Its flexibility allows users to customize analyses in numerous ways, making it a preferred tool for data scientists and statisticians who need advanced modeling techniques. The vast amount of packages available extends Rβs capabilities, covering everything from time series analysis to machine learning implementation.
R's strength lies in its community. Users benefit from a wealth of shared resources, tutorials, and forums. On the flip side, the learning curve can be steep for those not familiar with programming. For beginners, it might feel like a labyrinth at first glance.
Excel
Excel is often considered the most familiar statistical tool since itβs widely used across different industries. Itβs not solely a spreadsheet program anymore; with its built-in functions and capabilities for analysis, it's surprisingly versatile for basic statistical computing. Excel offers features like pivot tables and graphing tools that allow users to visualize data dynamically.
The accessibility of Excel makes it an appealing option. Many professionals already possess some level of competency with it. Nevertheless, Excel has limitations, especially when handling large datasets or conducting sophisticated analyses. Itβs great for quick calculations or small-scale analyses but doesnβt replace more specialized software for in-depth statistical work.
Choosing the Right Tools
Selecting the right statistical software depends greatly on the specific needs of the project. Here are some considerations:
- Budget: Consider what financial resources are available for purchasing software. Options like R are free, while SPSS usually requires a license that can be quite costly.
- Complexity of Analysis: If advanced analytics are needed, tools like R provide more versatility than Excel or SPSS.
- User Expertise: Evaluate the skill level of the users. While Excel is easy to use, R may require more technical knowledge. Assess how much time can be devoted to learning new software.
- Type of Data: The kind of data being analyzed can also dictate choice. For large-scale data analysis, SPSS or R would be more suitable compared to Excel.
In short, investing time in choosing the right software can yield dividends down the line, transforming how businesses understand and use their data.
Applications of Business Statistics
In the landscape of modern business, the significance of data cannot be overstated. Business statistics provide a framework for making sense of vast amounts of information, transforming raw data into actionable insights. Understanding the applications of business statistics is paramount for professionals looking to sharpen their decision-making skills and drive organizational performance. Whether itβs gauging market trends, predicting financial outcomes, or improving operational processes, every touchpoint of business benefits from applying statistical principles effectively.
Market Research
Market research stands as a cornerstone of any successful business strategy. Using business statistics, companies can delve into customer preferences, market demands, and competitive landscapes. By employing surveys and focus groups, businesses generate quantitative and qualitative data that can be statistically analyzed to identify trends and gaps in the market. This analysis helps organizations tailor their products and services to meet consumer needs, thereby boosting customer satisfaction and loyalty.
For instance, consider a fictional beverage company that plans to launch a new flavor. Through targeted surveys, they assess consumer interest and preferences. Results might reveal that 65% prefer fruity flavors with less sugar. This data can direct product development and marketing strategies, ensuring the new product aligns with consumer desires.
In essence, the application of statistics in market research molds the companyβs offerings, minimizes the risk of product failure, and ultimately leads to a better bottom line.
Financial Forecasting
Financial forecasting uses statistical techniques to predict future financial outcomes based on historical data. This application is vital for making informed budgeting and investment decisions. By analyzing trends in pricing, market volatility, and consumer spending, businesses can create robust financial models.
For example, a retail chain might analyze past sales during the holiday season, adjusting for variables like economic conditions and social trends. Utilizing techniques such as regression analysis, the chain can forecast expected sales for the upcoming season. This allows for effective inventory management, reducing the risk of overstock or stockouts.
In the realm of finance, accuracy is critical. By grounding forecasts in solid statistical methodologies, businesses can improve fiscal stability and strategic planning. In this case, employing business statistics not only helps manage risk but also lays the groundwork for sustainable growth.
Operational Efficiency
Operational efficiency hinges on the ability to optimize processes while minimizing waste. Here, business statistics serve as a tool for identifying inefficiencies and implementing improvements. By tracking performance metrics, organizations can analyze workflows, employee productivity, and resource utilization.
Take, for instance, a manufacturing plant aiming to reduce production time. By collecting data on output cycles and delays, statistical tools can identify bottlenecks and areas for improvement. These insights enable decision-makers to restructure operations and enhance overall productivity.
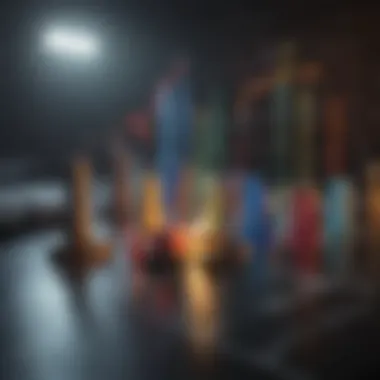
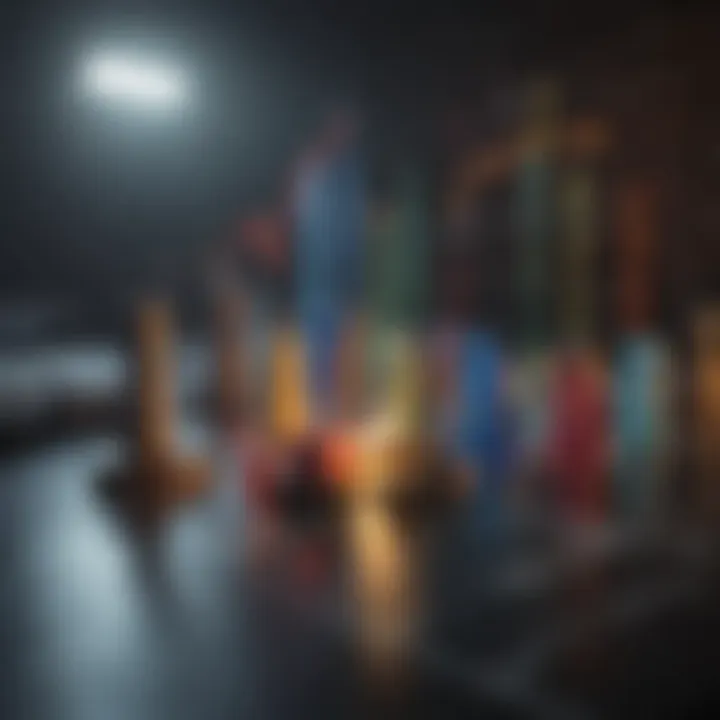
Additionally, statistical quality control techniques ensure products meet established standards while identifying areas requiring attention before issues escalate. Simply put, the integration of statistics into operations empowers businesses to make data-driven decisions that enhance performance, ensure quality, and ultimately save costs.
"In an age overwhelmed with data, the precision of statistical applications shapes the trajectory of business success."
In summary, the applications of business statistics are pivotal in creating a well-rounded approach to market research, financial forecasting, and operational efficiency. Each application enriches the decision-making process and elevates the strategic posture of an organization in a competitive landscape.
Challenges in Business Statistics
The landscape of business statistics is marked by complexities and nuances that can often prove daunting. Addressing challenges in business statistics is not just a matter of academic rigor; itβs about wielding data responsibly and making informed decisions. Such challenges are multi-faceted and can significantly impact the accuracy and utility of statistical findings.
In this section, we will navigate through critical challenges, examining how they affect data reliability and integrity while uncovering methods to mitigate common pitfalls. Understanding these challenges is essential for students, researchers, educators, and professionals who wish to harness the power of statistical analysis effectively.
Data Reliability and Validity
When diving into data analysis, reliability and validity emerge as cornerstones of good statistics. Reliability refers to the consistency of a measure; if you were to repeat the same measurement under unchanged conditions, you would arrive at similar results. Validity, on the other hand, assesses whether the tool is measuring what it is actually intended to measure.
- Data Sources: Understanding where data comes from is crucial. Data gathered from unreliable sources can compromise overall results. For example, relying solely on self-reported surveys can lead to skewed data because participants may misreport their habits or opinions.
- Sample Size: The number of observations can also impact reliability. A small sample size often cannot capture the variations necessary for a true reflection of the entire population, leading to results that might mislead.
- Statistical Tools: Utilizing robust statistical tools can help clarify whether data accurately represents its intended phenomena. Tools like SPSS or R assist in analyzing variance and checking reliability coefficients.
Ensuring data reliability and validity helps businesses make decisions based on sound evidence, rather than acting on flukes or misinterpretations that can arise from misleading data.
Overcoming Bias
Bias in data collection can pervert outcomes and lead to misguided strategies. Bias can come in many forms, whether it's selection bias, response bias, or confirmation bias, among others. Recognizing and mitigating these biases is imperative for maintaining the integrity of statistical analyses.
- Selection Bias: This occurs when the sample isnβt representative of the population it's drawn from. For instance, conducting an online survey where only certain demographics participate can yield results that don't reflect the broader population.
- Response Bias: Participants might not give genuine responses due to various factors, including social desirability. For example, a survey on political opinions might be skewed if people feel pressured to align with popular opinions.
- Confirmation Bias: Sometimes, statisticians might unconsciously seek out or favor information that confirms their prior beliefs, leading to skewed interpretations of data.
To combat these biases, practitioners can employ techniques such as randomized sampling, awareness training for survey administrators, and transparent reporting of methodologies. A commitment to ethical standards in research creates a foundation for credible results that can significantly inform business strategies.
"In statistics, the findings can only be as good as the data collected. Bad data leads to bad decisions."
Through a keen understanding of data reliability, validity, and bias, professionals can navigate the murky waters of statistical analysis. Tackling these challenges paves the way for more trustworthy statistics, ultimately enabling stronger decision-making grounded in fact rather than conjecture.
Ethics in Business Statistics
The significance of ethics in business statistics cannot be overstated. In a world where data informs nearly every decision made by businesses and organizations, ethical considerations become paramount. By establishing a solid ethical framework, companies not only uphold their integrity but also enhance their reputation. This, in turn, breeds trust among consumers, partners, and stakeholders. When individuals or entities trust an organizationβs handling of data, it opens doors to more fruitful collaborations and long-term relationships.
Understanding and adopting ethical principles safeguards the credibility of statistical findings. The impact of data on decision-making processes is profound; thus, it is critical to ensure that the collection, analysis, and presentation of data adhere to high ethical standards. This approach minimizes the risk of misinformation that can arise from improper practices and ultimately leads to more accurate assessments and conclusions.
Ethical Data Collection Practices
Through ethical data collection practices, businesses can gain valuable insights while maintaining respect for individuals' rights and privacy. Collecting data isn't merely about the numbers; it's about recognizing the people behind those numbers. Here are some fundamental principles to follow when gathering data:
- Informed Consent: It's essential to inform participants about the nature of the research, how their data will be used, and their right to withdraw at any time. Without proper consent, any findings might be questionable and unethical.
- Anonymity and Confidentiality: Protecting the identity of respondents is crucial. When individuals believe their responses are secure, they are more likely to provide honest and accurate information.
- Purpose Limitation: Use the data solely for the intended purpose. Misusing data for different objectives can lead to ethical and legal challenges.
- Avoiding Deceptive Practices: Misleading participants during data collection is not an option. Misrepresentation undermines the entire process and can nullify the results.
Adhering to these practices creates a strong foundation of ethical standards that can bolster credibility in research outcomes.
Transparency and Accountability
Emphasizing transparency and accountability in business statistics is essential. Organizations should be ready to uphold these tenets at every stage of statistical analysis. A transparent approach fosters trust and encourages open dialogue about the methodologies used in gathering and interpreting data.
- Documentation of Procedures: Keeping detailed records of how data is collected and analyzed fosters a culture of accountability. This is especially true for audits, as it demonstrates that the organization follows accepted practices.
- Data Sharing and Accessibility: When appropriate, sharing data sets and methodologies with the public or peers is a solid step towards transparency. This enables others to verify results or even improve upon them.
- Addressing Errors and Misrepresentation: Should inaccuracies arise, responsible organizations should own up to the mistakes, take necessary corrective actions, and communicate transparently about the oversight. This honesty can mitigate potential fallout from errors in data reporting.
"Trust in business statistics is built on transparency and a commitment to ethical practices."
Future Trends in Business Statistics
The future of business statistics is not just a topic of interest; itβs a crucial facet of how organizations will operate in an increasingly data-driven world. Understanding what lies ahead in this domain helps businesses pivot towards strategies that capitalize on emerging trends. With the advancements in technology and a growing sea of data, organizations are in a position to leverage insights in innovative ways. The main focal points include big data and predictive analytics, both of which are reshaping the statistical landscape.
Big Data and Its Implications
Big data refers to the vast volumes of data generated every moment, from customer transactions to social media interactions. This phenomenon poses both challenges and opportunities for businesses.
The sheer scale and variety of the data necessitate advanced statistical methods for effective analysis.
- Enhanced Decision-Making: Big data allows companies to make informed decisions based on real-time insights. For instance, businesses can tap into customer behaviors, preferences, and trends to tailor their offerings more precisely.
- Challenges in Data Management: Handling big data requires reliable tools and methods. Companies must juggle the complexities of data storage and retrieval, ensuring that they maintain high standards of data integrity.
- Ethical Considerations: With large amounts of sensitive data at hand, organizations must tread carefully concerning ethical standards. Transparency in how data is collected and utilized is vital to maintaining consumer trust, which is essential in a competitive market.
"The data deluge has paved new roads for decision makers, but steering through it requires skill and integrity."
Investing in robust computational infrastructure and data analysis software becomes a necessity rather than a luxury. This aspect of big data cannot be overlooked, as it determines the organization's ability to adapt and thrive.
The Rise of Predictive Analytics
Predictive analytics utilizes statistical algorithms and machine learning techniques to identify the likelihood of future outcomes based on historical data. This approach is gaining traction across a variety of sectors, including healthcare, finance, and marketing.
Key elements that underscore its significance include:
- Proactive Strategy Development: Instead of merely reacting to past performance or trends, businesses can anticipate future market movements and customer needs. For example, a retail company may use predictive analytics to forecast inventory requirements, thereby enhancing operational efficiency.
- Risk Management: Understanding risk before it materializes is invaluable. Financial institutions employ predictive analytics to assess credit scores and detect fraud, ultimately saving money and protecting their assets more effectively.
- Personalization at Scale: Predictive models empower companies to tailor their customer experiences dynamically. Think about how streaming services like Netflix suggest shows based on a userβs viewing habits; this is all thanks to sophisticated predictive analytics.
The implications of these trends are far-reaching, affecting how businesses strategize and compete in their respective markets. Itβs no longer sufficient for companies to simply collect data; the ability to harness this data effectively will be the differentiating factor between thriving and merely surviving in the coming years.